Deep reinforcement learning-based complex terrain self-adaptive motion control method for hexapod robot
A hexapod robot and motion control technology, applied in the direction of adaptive control, general control system, control/regulation system, etc., can solve the problem of hexapod robot falling to the ground, achieve fast convergence of neural network, speed up training, improve The effect on survival
- Summary
- Abstract
- Description
- Claims
- Application Information
AI Technical Summary
Problems solved by technology
Method used
Image
Examples
Embodiment 1
[0024] Such as figure 1 As shown, the present invention provides a hexapod robot complex terrain adaptive motion control method based on deep reinforcement learning, comprising the following steps:
[0025] S1. The terrain information of the complex environment is obtained by the quadcopter through the overlooking camera, and according to the terrain information of the environment, the current position of the hexapod robot is used as the starting point, an end point is set, the trajectory is planned, and passed to the hexapod robot;
[0026] S2. The hexapod robot obtains the environmental photos around the body through the RGB camera installed on the body, denoted as D1, and obtains the current state information of the body through the sensors installed on the body of the hexapod robot, including linear velocity, angular velocity, and hexapod robot. The attitude in three-dimensional space represents the quaternion, and the angle of each leg joint degree of freedom, denoted as ...
PUM
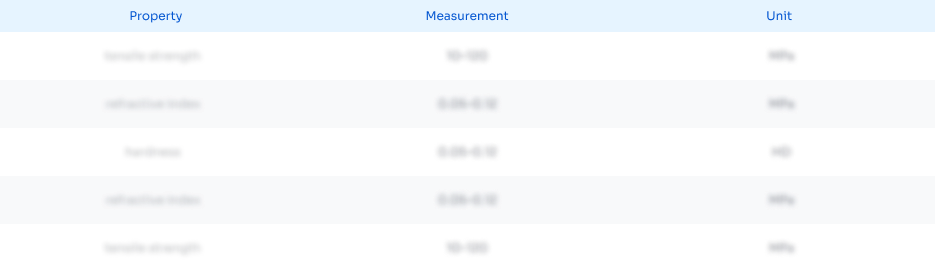
Abstract
Description
Claims
Application Information
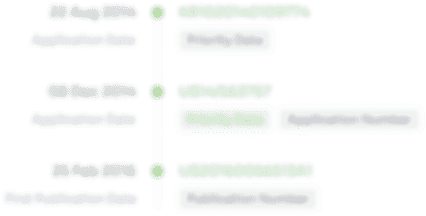
- R&D Engineer
- R&D Manager
- IP Professional
- Industry Leading Data Capabilities
- Powerful AI technology
- Patent DNA Extraction
Browse by: Latest US Patents, China's latest patents, Technical Efficacy Thesaurus, Application Domain, Technology Topic, Popular Technical Reports.
© 2024 PatSnap. All rights reserved.Legal|Privacy policy|Modern Slavery Act Transparency Statement|Sitemap|About US| Contact US: help@patsnap.com