Multi-fault-mode high-performance binary-classifier of large wind power gearbox
A wind power gearbox and two-classifier technology, applied in character and pattern recognition, instruments, computer parts, etc., can solve the problem of reducing the generalization and promotion ability of the classifier, amplifying the negative effects of noise or outliers, and estimating the statistical characteristics of samples. And other issues
- Summary
- Abstract
- Description
- Claims
- Application Information
AI Technical Summary
Problems solved by technology
Method used
Image
Examples
Embodiment 1
[0046] Multiple Failure Mode Classification
[0047] The spatial distribution of the fault mode characteristics of the three typical wind turbine gearboxes in normal state, gear tooth damage and frame motion is as follows: image 3 shown. Construct the training set using multimodal class feature samples:
[0048] [I w (k,l)] n =W(k,l)I n (x 0 - +k,y 0 - +l),
[0049] [I w (k,l)] g =W(k,l)I g (x 0 - +k,y 0 - +l),
[0050] ]I w (k,l)] l =W(k,l)I l (x 0 - +k,y 0 - +l).
[0051] where [·] n ,[·] g as well as[·] l are the feature training sets of the normal state, gear tooth damage and machine base transmission mode, respectively, I n (·,·), I g (·,·) and I l (·,·) are the size M respectively 1 × M 2 The spatial feature distribution image of .
[0052] First, the FLSA-SVM classification model is initialized, and the kernel function is selected as Radial Basis Function (RBF). The initial model is then tuned using a one-to-...
Embodiment 2
[0060] Multiple failure mode classification
[0061] Combining the training set and test set of the two modes of gear tooth damage and machine base loosening constitute the abnormal mode training set and test set, and use the FastICA algorithm to batch train two ICA feature extraction networks, thus forming [normal-abnormal Normal] feature extractor extracts two-dimensional quantized features of normal and abnormal patterns respectively. The number of training feature vectors is 20 and 40 respectively, and the same is true for testing feature vectors.
[0062] Two FLSA-SVM classifiers are trained by directly using the training samples of the four pattern categories of normal and abnormal, gear tooth damage and machine base looseness, and the kernel function still uses RBF. The classification test process is divided into two levels, the first level is used to identify normal and abnormal conditions, and the second level is used to identify tooth damage and frame loose mod...
PUM
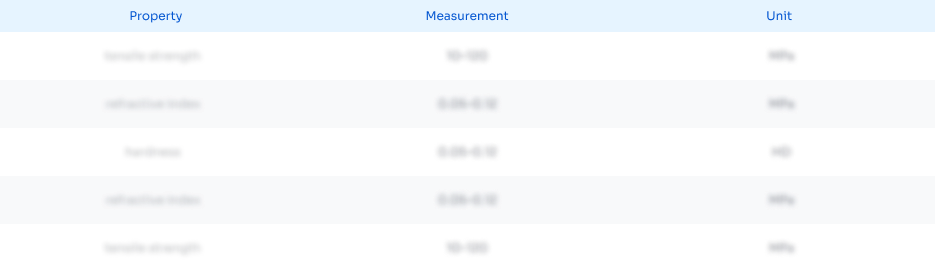
Abstract
Description
Claims
Application Information
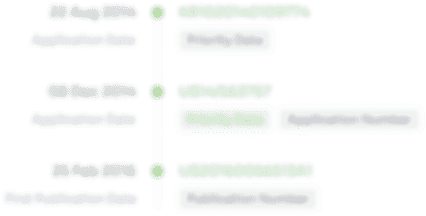
- R&D Engineer
- R&D Manager
- IP Professional
- Industry Leading Data Capabilities
- Powerful AI technology
- Patent DNA Extraction
Browse by: Latest US Patents, China's latest patents, Technical Efficacy Thesaurus, Application Domain, Technology Topic, Popular Technical Reports.
© 2024 PatSnap. All rights reserved.Legal|Privacy policy|Modern Slavery Act Transparency Statement|Sitemap|About US| Contact US: help@patsnap.com