Transfer and semi-supervised learning-based spatial estimation method for air quality index of non-city region
An air quality index and semi-supervised learning technology, applied in the field of machine learning, can solve problems such as inability to train models, scarcity, and labeled data that cannot cover all types of non-urban areas
- Summary
- Abstract
- Description
- Claims
- Application Information
AI Technical Summary
Problems solved by technology
Method used
Image
Examples
Embodiment Construction
[0059] The present invention will be further described below in conjunction with the accompanying drawings.
[0060] refer to Figure 1 ~ Figure 3 , a method for spatial estimation of air quality index in non-urban areas based on transfer semi-supervised learning, characterized in that the method comprises the following steps:
[0061] (1) Based on the terrain distribution and the deployment of air quality monitoring stations, find auxiliary areas around the target area and construct auxiliary sample sets;
[0062] (2) Based on transfer learning technology, combined with labeled sample sets and auxiliary sample sets in the target area, train multiple regression models;
[0063] (3) Based on semi-supervised learning technology, using the unlabeled sample set in the target area, enhancing and fusing multiple regression models to obtain the final air quality index spatial estimation model.
[0064] Further, in the step (1), the steps of data preparation are as follows:
[0065...
PUM
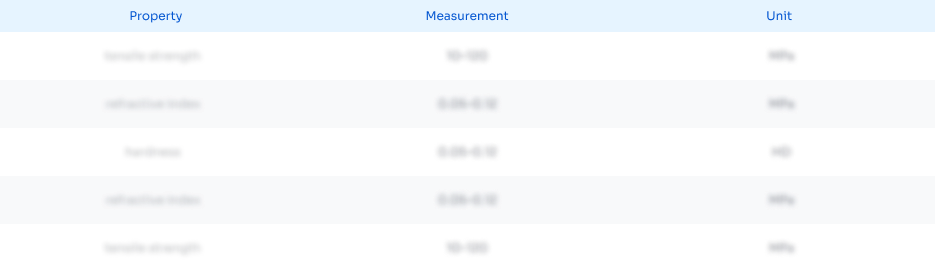
Abstract
Description
Claims
Application Information
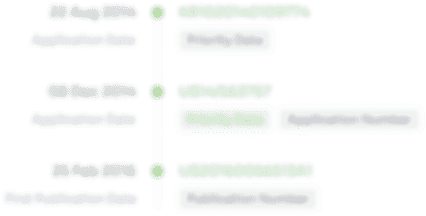
- R&D Engineer
- R&D Manager
- IP Professional
- Industry Leading Data Capabilities
- Powerful AI technology
- Patent DNA Extraction
Browse by: Latest US Patents, China's latest patents, Technical Efficacy Thesaurus, Application Domain, Technology Topic, Popular Technical Reports.
© 2024 PatSnap. All rights reserved.Legal|Privacy policy|Modern Slavery Act Transparency Statement|Sitemap|About US| Contact US: help@patsnap.com