Image classification method based on direction gradient histogram in combination with pseudo-reverse learning training stack self-encoder
A technology of directional gradient and autoencoder, which is applied in the direction of instruments, biological neural network models, character and pattern recognition, etc., can solve the problems of consuming a lot of manpower and time, achieve fast training time, short training time, and reduce related features Effect
- Summary
- Abstract
- Description
- Claims
- Application Information
AI Technical Summary
Problems solved by technology
Method used
Image
Examples
example
[0057] In order to prove that the present invention is practical, we use the commonly used data sets for machine learning to test the performance of the model. And compared with related models.
[0058] The database used in the experiment is THE MNIST DATABASE of handwritten digits. MNIST is now recognized by the industry as a standard dataset with excellent performance for detection and classification algorithms. We test the model performance of the present invention using MNIST. MNIST created by Yann LeCun et al. contains 0-9 handwritten digital image data set. The data set contains a total of 70000 handwritten digital images, including 60000 training images and 10000 detection images. is aligned onto an image of 28×28=784 pixels. We use the classic machine learning, neural network model and the model of the present invention to compare based on the direction gradient histogram combined with the pseudo-inverse learning training stack autoencoder, and the results are shown ...
PUM
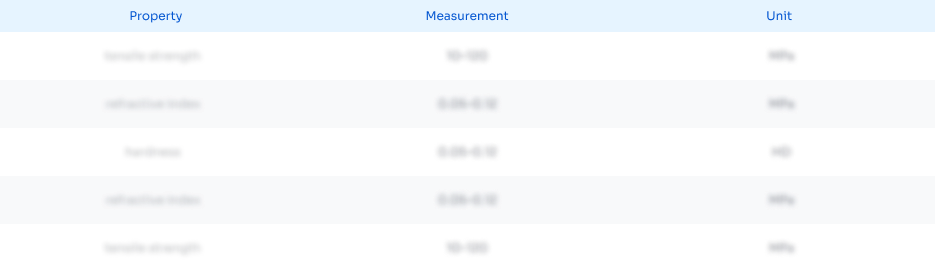
Abstract
Description
Claims
Application Information
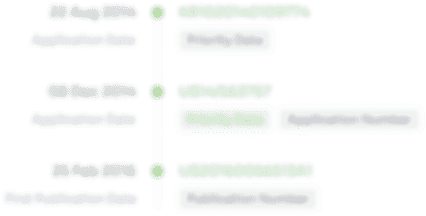
- R&D Engineer
- R&D Manager
- IP Professional
- Industry Leading Data Capabilities
- Powerful AI technology
- Patent DNA Extraction
Browse by: Latest US Patents, China's latest patents, Technical Efficacy Thesaurus, Application Domain, Technology Topic, Popular Technical Reports.
© 2024 PatSnap. All rights reserved.Legal|Privacy policy|Modern Slavery Act Transparency Statement|Sitemap|About US| Contact US: help@patsnap.com