Deep learning prediction method based on combined framework of plurality of convolution neural networks
A convolutional neural network and prediction method technology, applied in the field of deep learning prediction based on multiple convolutional neural networks combined with architecture, can solve the problems of time-consuming engineers, limited data storage scale, poor neural network performance, etc., and achieve resource utilization Improve efficiency, improve prediction accuracy, and alleviate the effect of overfitting
- Summary
- Abstract
- Description
- Claims
- Application Information
AI Technical Summary
Problems solved by technology
Method used
Image
Examples
Embodiment Construction
[0025] In order to make the object, technical solution and advantages of the present invention more clear, the present invention will be further described in detail below in conjunction with the examples. It should be understood that the specific embodiments described here are only used to explain the present invention, not to limit the present invention.
[0026] The present invention is based on a deep learning prediction method based on a novel multiple convolutional neural network combined architecture, which can effectively utilize multi-dimensional time series information and produce stable and accurate results when the amount of data is not very sufficient.
[0027] like figure 1 As shown, the deep learning prediction method based on multiple convolutional neural networks combined with the architecture provided by the embodiment of the present invention includes the following steps:
[0028] S101: Transform the data;
[0029] S102: Prepare a training data set, a veri...
PUM
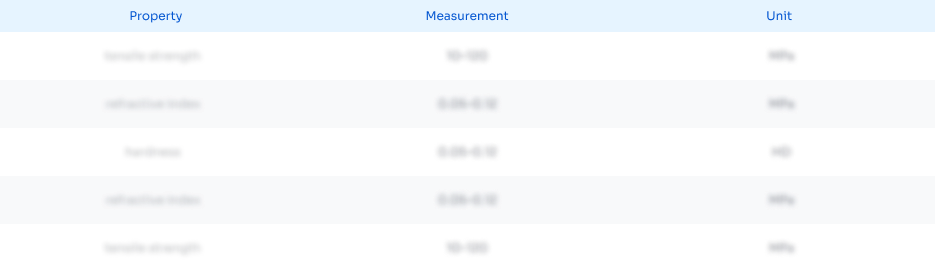
Abstract
Description
Claims
Application Information
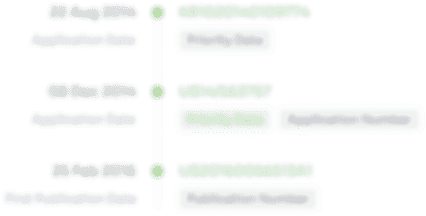
- Generate Ideas
- Intellectual Property
- Life Sciences
- Materials
- Tech Scout
- Unparalleled Data Quality
- Higher Quality Content
- 60% Fewer Hallucinations
Browse by: Latest US Patents, China's latest patents, Technical Efficacy Thesaurus, Application Domain, Technology Topic, Popular Technical Reports.
© 2025 PatSnap. All rights reserved.Legal|Privacy policy|Modern Slavery Act Transparency Statement|Sitemap|About US| Contact US: help@patsnap.com