A fiber grating wavelength demodulation method and device based on deep learning
A fiber grating and wavelength demodulation technology, applied in biological neural network models, design optimization/simulation, neural architecture, etc., can solve problems such as slow training speed, cumbersome neural network parameter selection, local optimization and overfitting, etc. Achieve the effect of improving accuracy, effective high-speed high-precision demodulation, and high flexibility
- Summary
- Abstract
- Description
- Claims
- Application Information
AI Technical Summary
Problems solved by technology
Method used
Image
Examples
Embodiment 1
[0071] please see figure 1 , a kind of fiber grating demodulation method based on deep learning provided by the present invention comprises the following steps:
[0072] Step 01: Obtain the wave peak data of the fiber grating wavelength demodulator, fit these data with traditional methods, and obtain their Gaussian function parameters as the output sample Y;
[0073] Step 02: Use the function expression determined by Gaussian parameters to randomly generate L data points as input samples in Generate m training samples according to this method;
[0074] Step 03: Define a neural network with n layers {h 1 , h 2 ,...,h n}, the output function of each layer is expressed as h i (x)=g(θ i x); where g(x) represents a nonlinear activation function, different activation functions are allowed between different neural network layers, θ i Represents the parameter matrix of the i-th layer, and x represents the input vector of neurons in the corresponding layer;
[0075] Step 04:...
Embodiment 2
[0092] This embodiment belongs to the device embodiment, and belongs to the same technical concept as the method embodiment in the above-mentioned embodiment 1. For the content not described in detail in this embodiment, please refer to the method embodiment 1.
[0093] Such as figure 2 As shown, a kind of fiber grating wavelength demodulation device based on deep learning described in the present invention comprises:
[0094] An analysis unit is used to analyze the peak data received by the fiber grating wavelength demodulator and generate training samples;
[0095] an initialization unit for initializing the neural network;
[0096] The first processing unit is used to collect training samples and perform BatchNormalization processing on each layer of neurons;
[0097] The second processing unit is used to perform Dropout processing on each layer of neurons, and introduce the L2 norm for regularization, and use the stochastic gradient descent method to update the network we...
PUM
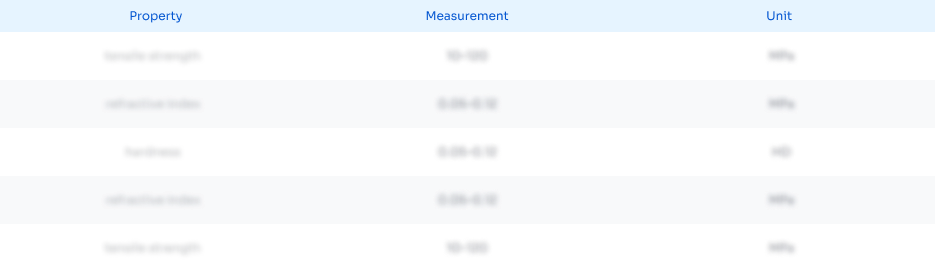
Abstract
Description
Claims
Application Information
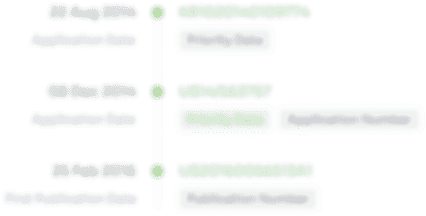
- R&D Engineer
- R&D Manager
- IP Professional
- Industry Leading Data Capabilities
- Powerful AI technology
- Patent DNA Extraction
Browse by: Latest US Patents, China's latest patents, Technical Efficacy Thesaurus, Application Domain, Technology Topic, Popular Technical Reports.
© 2024 PatSnap. All rights reserved.Legal|Privacy policy|Modern Slavery Act Transparency Statement|Sitemap|About US| Contact US: help@patsnap.com