Mobility prediction-based crowd sensing user recruitment method and system
A mobile crowd sensing and mobile user technology, applied in the field of crowd sensing user recruitment based on mobility prediction, can solve the problem of not conforming to the development trend of mobile crowd sensing, hindering the development of mobile crowd sensing, and wasting resources of participating mobile users and other issues, to achieve the effect of strong practical value, broad market prospects, and good privacy protection
- Summary
- Abstract
- Description
- Claims
- Application Information
AI Technical Summary
Problems solved by technology
Method used
Image
Examples
Embodiment 1
[0056] figure 1 It is a flowchart of an embodiment of the mobility prediction-based crowd sensing user recruitment method of the present invention, such as figure 1 As shown, the group intelligence sensing user recruitment method includes:
[0057] Step 101, sending a mobile crowd sensing task, the mobile crowd sensing task including budget, target location, task start time and task end time.
[0058] Step 102, obtaining individual expectation values of multiple mobile users, where the individual expectation values represent the probability that the mobile users arrive at the target location on time.
[0059] The personal expectation value is sent by the mobile device of the mobile user, and the specific calculation method of the personal expectation value is:
[0060] Obtain personal spatiotemporal trajectory information of mobile users; the personal spatiotemporal trajectory information includes: points of interest, time of entering said point of interest and time of l...
Embodiment 2
[0077] Embodiment 2 provides a mobility prediction-based crowd sensing user recruitment method, which specifically includes:
[0078] (1) In the preparation stage, mobile users collect their own spatiotemporal trajectory information, and construct a personal spatiotemporal semi-Markov mobility prediction model. A spatio-temporal trajectory is a collection of multiple spatio-temporal information triples , where l represents the point of interest, ts and te represent the time of entering and leaving the point of interest. According to the set of spatio-temporal information, the first core formula of the semi-Markov model can be obtained according to statistics:
[0079]
[0080] Among them, Z u (i, j, T) represents the probability that a mobile user transfers directly from point of interest i to point of interest j within T time, S u Indicates the sequence of POIs visited by mobile users, and the access time of POIs visited by mobile users. Obviously, the next POI visited b...
PUM
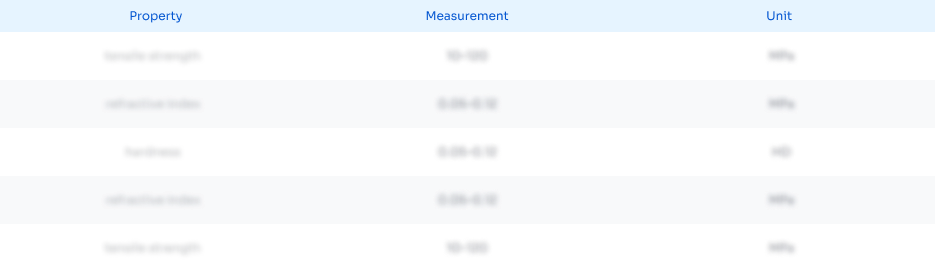
Abstract
Description
Claims
Application Information
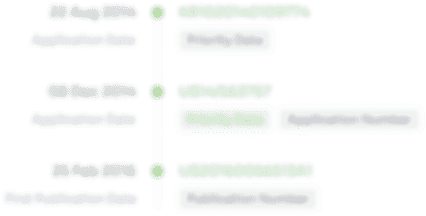
- R&D Engineer
- R&D Manager
- IP Professional
- Industry Leading Data Capabilities
- Powerful AI technology
- Patent DNA Extraction
Browse by: Latest US Patents, China's latest patents, Technical Efficacy Thesaurus, Application Domain, Technology Topic, Popular Technical Reports.
© 2024 PatSnap. All rights reserved.Legal|Privacy policy|Modern Slavery Act Transparency Statement|Sitemap|About US| Contact US: help@patsnap.com