Hydroelectric generating set fault diagnosis method and system based on DdAE (Difference Differential Algebraic Equations) deep learning model
A hydroelectric unit and fault diagnosis technology, applied in neural learning methods, biological models, computing models, etc., can solve cumbersome manual work and other problems, achieve the effects of improving anti-noise ability, avoiding gradient explosion, and improving feature learning ability
- Summary
- Abstract
- Description
- Claims
- Application Information
AI Technical Summary
Problems solved by technology
Method used
Image
Examples
Embodiment 1
[0045] The embodiment of the present invention is based on the fault diagnosis method flow of the deep denoising autoencoder (Deep denoising Autoencoder, abbreviated as DdAE) deep learning model is as follows figure 1 shown. Include the following execution steps:
[0046] In step 201, a data set is obtained, and n groups of data blocks are extracted from the data set as training data for the DdAE network model.
[0047] Taking the hydroelectric generating unit as an example, in step 201, the original data of the vibration of the hydroelectric unit is used as the input sample set x, and normalized processing is performed before being used as training data, even if the processed data is distributed according to the distribution ratio of the original data Between -1 and 1, a new input sample set x' is obtained; then the normalized sample set x' is equally divided into k groups of data blocks, considering the periodicity of the vibration fault of the hydroelectric unit, in order ...
Embodiment 2
[0090] The embodiment of the present invention is a composite solution that integrates many extended and preferred solutions in embodiment 1, wherein, refer to as Figure 6 The flow chart of the fault diagnosis method for hydroelectric units based on the DdAE deep learning model is shown. This embodiment mainly includes two processes of training and testing of the DdAE network model. The training process of the DdAE network model can be summarized as follows:
[0091] Step (1): Raw data preprocessing
[0092] In the embodiment of the present invention, the original data of the vibration of the hydroelectric unit is used as the input sample set x, and the normalization process is first adopted, even if the processed data is distributed between -1 and 1 according to the distribution ratio of the original data, a new input sample set is obtained x'; and then divide the normalized sample set x' into k groups of data blocks. Considering the periodicity of vibration faults of hydr...
Embodiment 3
[0158] The embodiment of the present invention also provides a hydroelectric unit fault diagnosis system based on the DdAE deep learning model, such as Figure 7 As shown, the system includes a training data processing module, a neural network model training module, a DdAE network model optimization module, a reconstruction feature vector generation module and a failure probability calculation module, and the above-mentioned modules are connected in sequence, specifically:
[0159] The training data processing module is used to obtain the data set, and extract n groups of data blocks from the data set as the training data of the DdAE network model;
[0160] Neural network model training module, for setting up DdAE network model, and utilize described training data to train DdAE network model, obtain the connection weight of DdAE network model;
[0161] The DdAE network model optimization module is used to use the structural parameters of DdAE and the hyperparameters in the err...
PUM
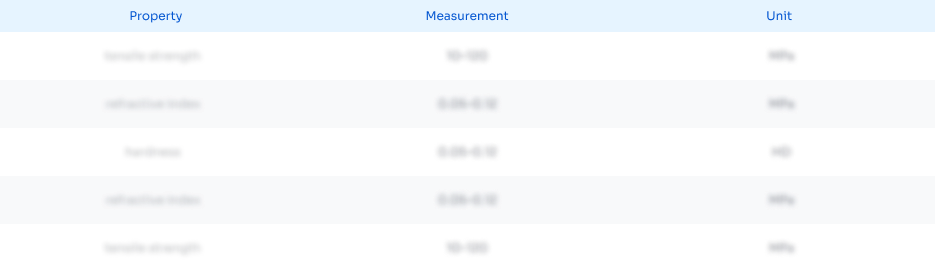
Abstract
Description
Claims
Application Information
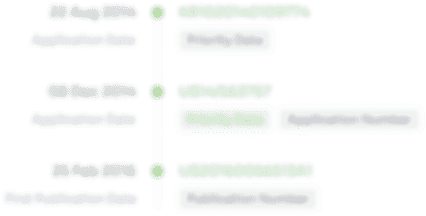
- R&D Engineer
- R&D Manager
- IP Professional
- Industry Leading Data Capabilities
- Powerful AI technology
- Patent DNA Extraction
Browse by: Latest US Patents, China's latest patents, Technical Efficacy Thesaurus, Application Domain, Technology Topic, Popular Technical Reports.
© 2024 PatSnap. All rights reserved.Legal|Privacy policy|Modern Slavery Act Transparency Statement|Sitemap|About US| Contact US: help@patsnap.com