CNN-DBN-based partial discharge fault diagnosis method
A fault diagnosis, BP network technology, applied in neural learning methods, biological neural network models, instruments, etc., to achieve the effect of improving accuracy
- Summary
- Abstract
- Description
- Claims
- Application Information
AI Technical Summary
Problems solved by technology
Method used
Image
Examples
Embodiment Construction
[0020] The present invention will be further described below in conjunction with the accompanying drawings. The following examples are only used to illustrate the technical solution of the present invention more clearly, but not to limit the protection scope of the present invention.
[0021] A partial discharge fault diagnosis method based on a CNN-DBN network, comprising the following steps:
[0022] Step 1. Construct a deep belief learning network based on a deep convolutional neural network and a restricted Boltzmann machine model.
[0023] The deep belief learning network includes several layers of convolutional neural networks, several layers of deep belief networks and several layers of BP networks connected in sequence. Combined with running speed and accuracy, after optimized design and verification, the optimal deep belief learning network is as follows: figure 1 As shown, it includes a 6-layer convolutional neural network (CNN network), a 4-layer unsupervised deep...
PUM
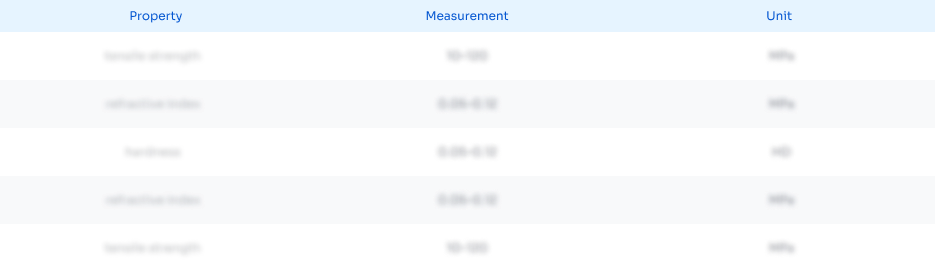
Abstract
Description
Claims
Application Information
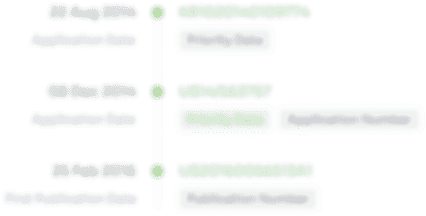
- R&D Engineer
- R&D Manager
- IP Professional
- Industry Leading Data Capabilities
- Powerful AI technology
- Patent DNA Extraction
Browse by: Latest US Patents, China's latest patents, Technical Efficacy Thesaurus, Application Domain, Technology Topic, Popular Technical Reports.
© 2024 PatSnap. All rights reserved.Legal|Privacy policy|Modern Slavery Act Transparency Statement|Sitemap|About US| Contact US: help@patsnap.com