SAR image target recognition method based on multilayer probability statistics model
A probabilistic statistical model and target recognition technology, applied in the field of SAR image target recognition based on multi-layer probabilistic statistical model, can solve the non-negative feature extraction method that cannot obtain effect, does not use category information, and does not involve multi-layer probabilistic statistical model etc. to achieve the effect of reducing the complexity of network parameters, improving the performance and stability of target recognition, and good representation ability
- Summary
- Abstract
- Description
- Claims
- Application Information
AI Technical Summary
Problems solved by technology
Method used
Image
Examples
Embodiment 1
[0026] The common point of some existing methods for SAR image feature extraction is that when the input data is non-negative, the obtained global variables and latent variables still have negative values, while the pixels in the SAR image data are all positive values, so these The features extracted by the method cannot be well explained physically.
[0027] In addition, the existing method of approximately decomposing the input matrix into a dictionary and a non-negative weighted combination of hidden variables can extract the non-negative features inside the SAR image and increase the performance of SAR image target recognition, but a single-layer feature extraction method, The structure of the mined information is relatively simple.
[0028]In terms of modeling methods, traditional deep networks such as Deep Belief Network (DBN) use binary data modeling for hidden variables, and have limited modeling capabilities for other forms of data. The layer stacking strategy trains...
Embodiment 2
[0040] The SAR image target recognition method based on the multi-layer probability statistical model is the same as embodiment 1, the parameters of the Poisson gamma belief network are initialized in the step (2), that is, the parameters of the multi-layer probability statistical model are initialized, and the initialized variables have the global value of the model parameters, hidden variable parameters, and hyperparameters of each prior distribution, such as Φ (l,C) ,θ (l,C) ,r C ,γ 0 C ,c 0 C , η (l,C) ,p (2 ,C) ,c (3,C) ,a 0 C ,e 0 C ,b 0 C ,f 0 C ,K l C , hyperparameter a 0 C , b 0 C , e 0 C , f 0 C No need to update, other parameters need to be updated in the process of model training and testing, in this example for the global variable Φ (l,C) and latent variable θ (l,C) The initialization of includes the following steps:
[0041] Layer-l global variables for objects of class C Initialization proceeds as follows:
[0042] φ a (l,C) ~Di...
Embodiment 3
[0051] The SAR image target recognition method based on the multi-layer probability statistical model is the same as embodiment 1-2, the training multi-layer probability statistical model in step 3, see figure 2 , including the following steps:
[0052] (3a) Input various training samples, and set the number of network layers, the dimensions of the input layer and each hidden layer, and the number of iterations M of various training samples 1 .
[0053] (3b) Calculate each layer augmentation matrix, intra-layer augmentation matrix and inter-layer augmentation matrix in the process of uplink Gibbs sampling: use the Naive Bayesian criterion to independently train the multi-layer probability statistics model for each type of training data , in each iteration of various multi-layer probability statistical models, starting from the bottom layer, training layer by layer from the lower layer to the upper layer, and calculating the augmented matrix and intra-layer Augmented matrix an...
PUM
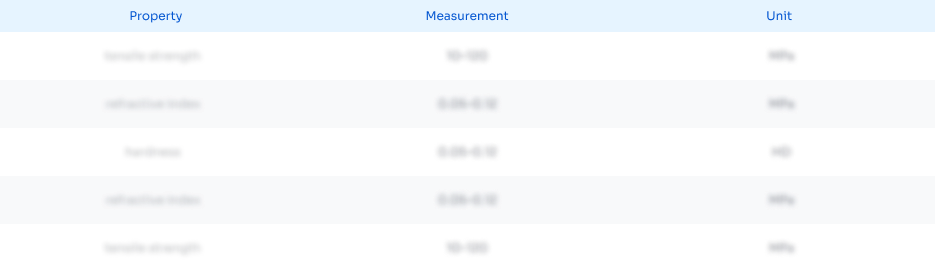
Abstract
Description
Claims
Application Information
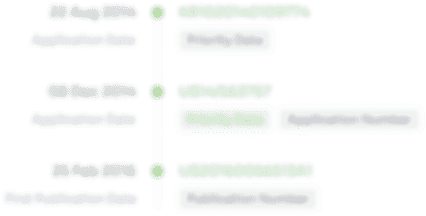
- R&D Engineer
- R&D Manager
- IP Professional
- Industry Leading Data Capabilities
- Powerful AI technology
- Patent DNA Extraction
Browse by: Latest US Patents, China's latest patents, Technical Efficacy Thesaurus, Application Domain, Technology Topic, Popular Technical Reports.
© 2024 PatSnap. All rights reserved.Legal|Privacy policy|Modern Slavery Act Transparency Statement|Sitemap|About US| Contact US: help@patsnap.com