Method for establishing DO prediction model based on optimized BP neural network
A BP neural network and prediction model technology, applied in biological neural network models, neural learning methods, neural architectures, etc., can solve problems such as failure to meet prediction requirements, failure to meet practical application requirements, results and actual output deviations, etc. , to achieve the effect of improving prediction accuracy, improving model performance, and facilitating precise control
- Summary
- Abstract
- Description
- Claims
- Application Information
AI Technical Summary
Problems solved by technology
Method used
Image
Examples
Embodiment 1
[0062] A method for establishing DO prediction model based on optimized BP neural network, including the following steps:
[0063] S1. Variable selection based on the goal of "aeration tank DO prediction".
[0064] Analyze the collected variables of the sewage treatment process related to the sewage treatment plant, and combine the actual situation of the sewage treatment plant to collect the influent F, the influent COD, the aeration volume Q, the aeration tank level LT, and the aeration tank sewage For variable data such as mud concentration MLSS, the correlation between each variable and the DO of the aeration tank is obtained through correlation analysis, and the factor variables with obvious correlation with the DO of the aeration tank are selected.
[0065] Through the correlation analysis between each variable and DO of the aeration tank from the perspective of the process and the data actually collected by the sewage treatment plant, the variables that have a significant impa...
Embodiment 2
[0115] Such as figure 1 , A method for establishing DO prediction model based on optimized BP neural network, including the following modeling and model evaluation steps:
[0116] 1. Through the control system of a sewage treatment plant to obtain the relevant data of the sewage treatment process, select all the data including 2017-03-03 07:00 to 2017-03-04 12:00 (data for this period (The lack of volume is relatively small), combined with the A2O process of sewage treatment, select the water intake F, the water COD, the aeration tank aeration Q, the aeration tank liquid level LT, and the aeration tank dissolved oxygen DO. Aeration tank sludge concentration MLSS and other variables. Using the Pearson correlation coefficient of formula (1) in Example 1, analyze the correlation between each variable and the DO of the aeration tank through correlation analysis, and select the variable that has a significant numerical correlation with the DO of the aeration tank—water intake F. Aer...
PUM
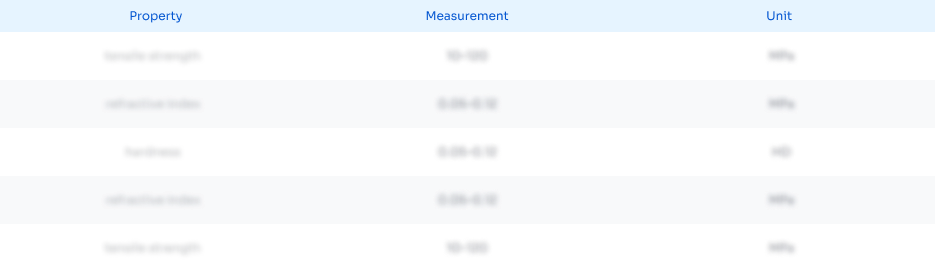
Abstract
Description
Claims
Application Information
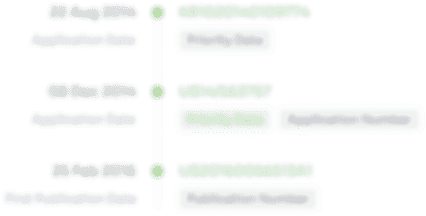
- R&D Engineer
- R&D Manager
- IP Professional
- Industry Leading Data Capabilities
- Powerful AI technology
- Patent DNA Extraction
Browse by: Latest US Patents, China's latest patents, Technical Efficacy Thesaurus, Application Domain, Technology Topic, Popular Technical Reports.
© 2024 PatSnap. All rights reserved.Legal|Privacy policy|Modern Slavery Act Transparency Statement|Sitemap|About US| Contact US: help@patsnap.com