Improved AdaBoost algorithm-based integrated deep belief network image identification method
A deep belief network and image recognition technology, applied in the field of deep learning and image recognition, can solve problems such as inability to process and recognize images, and achieve the effect of solving gradient dispersion and improving recognition rate.
- Summary
- Abstract
- Description
- Claims
- Application Information
AI Technical Summary
Problems solved by technology
Method used
Image
Examples
Embodiment 1
[0023] (1) Preparation of training and test sets: Take 1000 images of each class from the MNIST dataset to form a training set, and 100 images of each class to form a test set; take 500 images of each class from the USPS dataset to form a training set, and 50 images of each class form a test set set; 300 images of each class are taken from the ETH-80 dataset to form a training set, and 100 images of each class form a test set.
[0024] (2) Construction of DBN model: In the implementation of the method, we consider the following four DBN network structure models: DBN(4)[400,256,100,64], DBN(6)[400,256,196,144,100,64] and AdaBoost+DBN(4){[400,256,100 , 64], [256, 144, 100, 64], [100, 81, 81, 60], [225, 196, 144, 100]}, where the data in the square brackets of each model is the number of hidden layer units of the model, and the number of data represents the hidden layer of the model. layers.
[0025] (3) Use the normalized image vector as the input of the model to train each net...
PUM
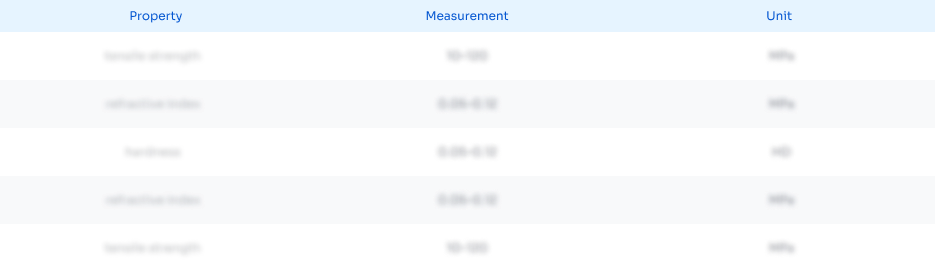
Abstract
Description
Claims
Application Information
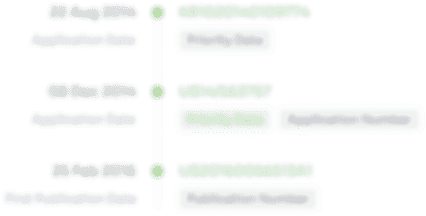
- R&D Engineer
- R&D Manager
- IP Professional
- Industry Leading Data Capabilities
- Powerful AI technology
- Patent DNA Extraction
Browse by: Latest US Patents, China's latest patents, Technical Efficacy Thesaurus, Application Domain, Technology Topic, Popular Technical Reports.
© 2024 PatSnap. All rights reserved.Legal|Privacy policy|Modern Slavery Act Transparency Statement|Sitemap|About US| Contact US: help@patsnap.com