Feature extraction and state recognition of one-dimensional physiological signal based on depth learning
A physiological signal and feature extraction technology, applied in the field of medical data processing, can solve problems such as low classification accuracy, unstable recognition effect, and manual feature extraction
- Summary
- Abstract
- Description
- Claims
- Application Information
AI Technical Summary
Problems solved by technology
Method used
Image
Examples
Embodiment Construction
[0092] The hardware and software environment used in the experiment of this embodiment are shown in Table 4-1:
[0093] Table 4-1
[0094]
[0095] data collection:
[0096] The experimental data is provided by Shanghai Jiaotong University emotional EEG database (SJTU Emotion EEGDataset, SEED) [, this database contains three types of emotional data (positive, negative, neutral) based on EEG signals. The data was collected from 15 subjects. Each experiment required each subject to watch 15 movie clips that could induce these three emotions. During the process of watching the movie clips, 62 channels of dry electrode EEG The cap collects the EEG signals of the subjects, each subject gets 15 sets of EEG signals in each experiment, and labels each set of EEG signals according to the descriptions of the subjects (positive is "+1", negative is " -1", neutral is "0"), there are 5 groups of "positive", 5 groups of "negative", and 5 groups of "neutral". Each subject performed th...
PUM
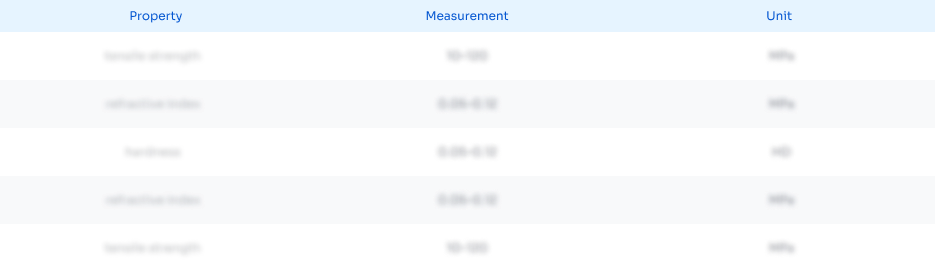
Abstract
Description
Claims
Application Information
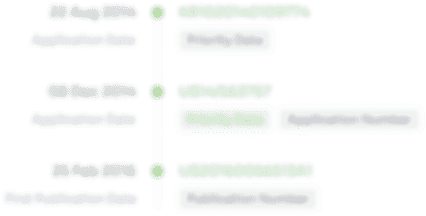
- R&D Engineer
- R&D Manager
- IP Professional
- Industry Leading Data Capabilities
- Powerful AI technology
- Patent DNA Extraction
Browse by: Latest US Patents, China's latest patents, Technical Efficacy Thesaurus, Application Domain, Technology Topic, Popular Technical Reports.
© 2024 PatSnap. All rights reserved.Legal|Privacy policy|Modern Slavery Act Transparency Statement|Sitemap|About US| Contact US: help@patsnap.com