Electrocardiogram signal classification and recognition method
A technology for classification and recognition, electrocardiogram signal, applied in the medical field, can solve the problems of limited nonlinear function fitting ability, limited nonlinear function fitting ability, etc., to achieve the effect of effective and more accurate classification and good social benefits
- Summary
- Abstract
- Description
- Claims
- Application Information
AI Technical Summary
Problems solved by technology
Method used
Image
Examples
Embodiment Construction
[0032] In this embodiment, the electrocardiographic signal classification and identification method is carried out according to the following steps,
[0033] (1) Obtain the waveform data of the two-lead electrocardiogram, and intercept the data whose length is 10 seconds according to the waveform data of the electrocardiogram as the original waveform data of the electrocardiogram;
[0034] (2) According to needs, the original ECG waveform data obtained in step (1) can be denoised. The denoising process uses a high-pass filter to remove baseline drift noise. When the noise is too high, use a low-pass Butterworth filter to remove noise interference. ;
[0035] (3) Set the number of nodes in the input layer, hidden layer, and output layer of the convolutional neural network, and randomly set the weights between nodes in adjacent layers; the typical structure of the convolutional neural network is as follows: figure 1 shown;
[0036] (4) To train the convolutional neural network...
PUM
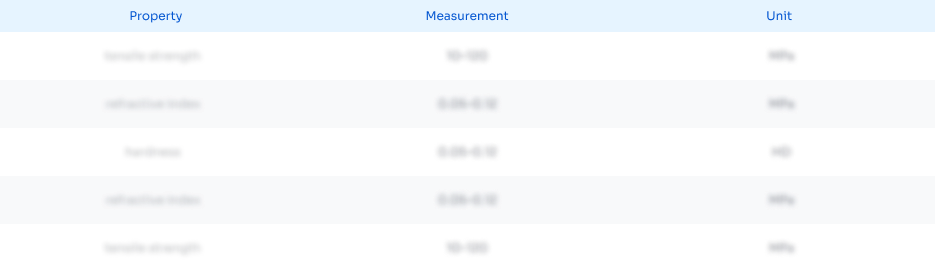
Abstract
Description
Claims
Application Information
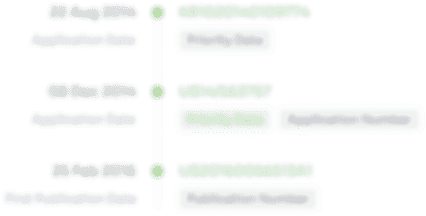
- R&D Engineer
- R&D Manager
- IP Professional
- Industry Leading Data Capabilities
- Powerful AI technology
- Patent DNA Extraction
Browse by: Latest US Patents, China's latest patents, Technical Efficacy Thesaurus, Application Domain, Technology Topic, Popular Technical Reports.
© 2024 PatSnap. All rights reserved.Legal|Privacy policy|Modern Slavery Act Transparency Statement|Sitemap|About US| Contact US: help@patsnap.com