Gaussian background modeling and recurrent neural network combined vehicle type classification method
A cyclic neural network and Gaussian background technology, applied in the field of computer machine vision classification, can solve the problems that the recognition accuracy needs to be improved and cannot overcome the false detection of the Gaussian mixture model.
- Summary
- Abstract
- Description
- Claims
- Application Information
AI Technical Summary
Problems solved by technology
Method used
Image
Examples
Embodiment Construction
[0031] The invention provides a vehicle classification method and system combining a Gaussian background modeling and a cyclic neural network, aiming at effectively and accurately classifying vehicle types in complex expressway scenes, and improving classification accuracy. The invention can be applied to occasions such as expressway monitoring systems and the like, and has good practicability. In the following, the present invention will be described in more detail and concretely with reference to the accompanying drawings and examples.
[0032] The first step is to model the mixed Gaussian background and extract moving objects. Such as figure 1 ,Specific steps are as follows:
[0033] 1. Initialize the highway background, first use the first n frames of continuous video stream images of the video to construct the highway background.
[0034] 2. Use K Gaussian distributions to approximate the gray value of each pixel in each frame of the image (the K value is generally 3-5...
PUM
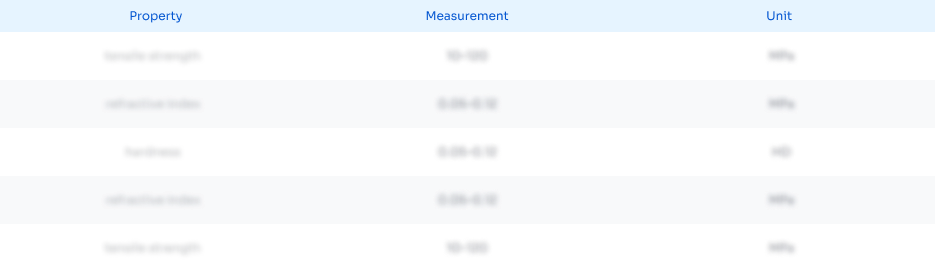
Abstract
Description
Claims
Application Information
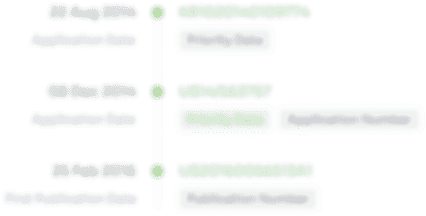
- R&D Engineer
- R&D Manager
- IP Professional
- Industry Leading Data Capabilities
- Powerful AI technology
- Patent DNA Extraction
Browse by: Latest US Patents, China's latest patents, Technical Efficacy Thesaurus, Application Domain, Technology Topic, Popular Technical Reports.
© 2024 PatSnap. All rights reserved.Legal|Privacy policy|Modern Slavery Act Transparency Statement|Sitemap|About US| Contact US: help@patsnap.com