Multiple kernel learning classification method based on noise probability function
A technology of probability function and classification method, applied in the direction of instruments, character and pattern recognition, computer parts, etc., can solve the problems of small amount of calculation, noise sensitivity, etc., to achieve small amount of calculation, solve noise-sensitive problems, robustness Good results
- Summary
- Abstract
- Description
- Claims
- Application Information
AI Technical Summary
Problems solved by technology
Method used
Image
Examples
Embodiment
[0062] In order to verify the correctness and effectiveness of this method, we use 6 UCI datasets for experiments. For each data set, 8 kernel functions (5 Gaussian kernel functions, 3 polynomial kernel functions) are used, as shown in Table 1 below:
[0063] Table 1 Information of UCI dataset
[0064] Datasets Samples Features Classes Balance-scale 567 4 2 Breast-cancer 569 32 2 Ionosphere 351 34 2 Blood-transfusion 748 5 2 Diabetic-retinopathy 1151 20 2 Pima-indians 768 8 2
[0065] At each noise level, the experiment was repeated 30 times on the data set, and the experimental results were the average of 30 experiments, as shown in Table 2 below:
[0066]
[0067]
PUM
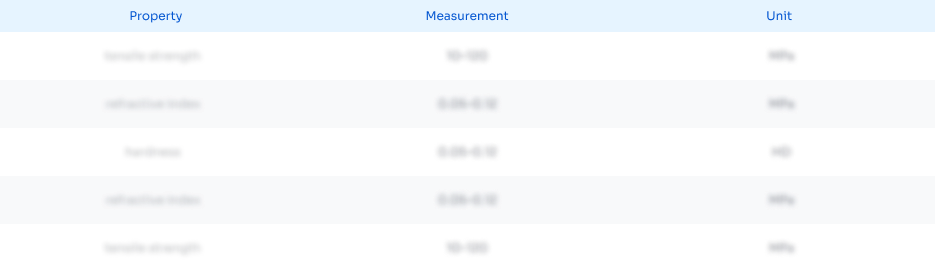
Abstract
Description
Claims
Application Information
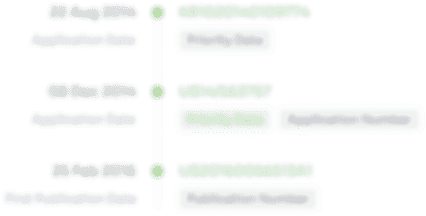
- R&D Engineer
- R&D Manager
- IP Professional
- Industry Leading Data Capabilities
- Powerful AI technology
- Patent DNA Extraction
Browse by: Latest US Patents, China's latest patents, Technical Efficacy Thesaurus, Application Domain, Technology Topic, Popular Technical Reports.
© 2024 PatSnap. All rights reserved.Legal|Privacy policy|Modern Slavery Act Transparency Statement|Sitemap|About US| Contact US: help@patsnap.com