Image Classification Method Based on Sparse Autoencoder and Support Vector Machine
A sparse auto-encoder and support vector machine technology, which is applied in the fields of instruments, genetic models, computer parts, etc., can solve the problem that the single-layer sparse auto-encoder is not easy to extract complete and deep abstract features, which affects the accuracy of image classification, The lack of robustness of features can achieve good generalization ability and scope of application, improve classification accuracy, and improve the effect of feature recognition.
- Summary
- Abstract
- Description
- Claims
- Application Information
AI Technical Summary
Problems solved by technology
Method used
Image
Examples
Embodiment Construction
[0019] Embodiments of the present invention are described in detail below, examples of which are shown in the drawings, wherein the same or similar reference numerals designate the same or similar elements or elements having the same or similar functions throughout. The embodiments described below by referring to the figures are exemplary only for explaining the present invention and should not be construed as limiting the present invention.
[0020] Such as figure 1 Shown, according to the image classification method based on sparse automatic encoder and support vector machine according to the present invention, comprise the following several steps:
[0021] S1: Obtain a training image set and a test image set;
[0022] S2: Construct a deep sparse autoencoder with multiple hidden layers, input the training image set, and train the deep sparse autoencoder until the training conditions are met.
[0023] The deep sparse autoencoder first needs to perform network training. The ...
PUM
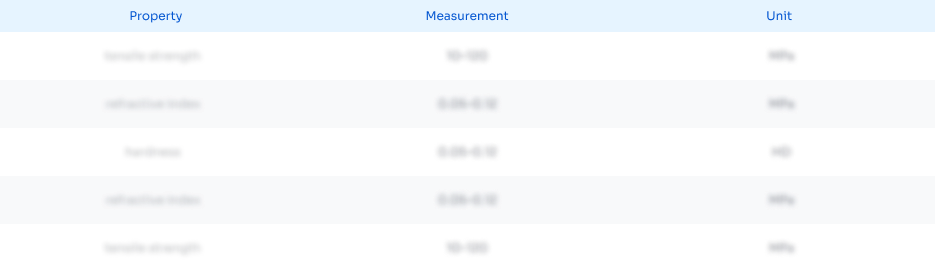
Abstract
Description
Claims
Application Information
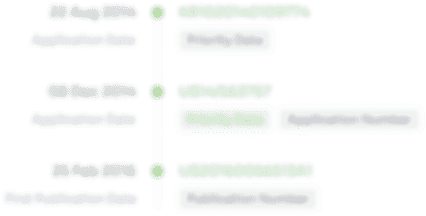
- R&D Engineer
- R&D Manager
- IP Professional
- Industry Leading Data Capabilities
- Powerful AI technology
- Patent DNA Extraction
Browse by: Latest US Patents, China's latest patents, Technical Efficacy Thesaurus, Application Domain, Technology Topic, Popular Technical Reports.
© 2024 PatSnap. All rights reserved.Legal|Privacy policy|Modern Slavery Act Transparency Statement|Sitemap|About US| Contact US: help@patsnap.com