Deep learning face verification method based on hybrid training
A face verification and deep learning technology, applied in the field of deep learning face verification based on hybrid training, can solve problems such as large amount of calculation, high model training time complexity, and large demand for training data, and achieve fast network training, The effect of fast network training and acquisition, efficient feature representation
- Summary
- Abstract
- Description
- Claims
- Application Information
AI Technical Summary
Problems solved by technology
Method used
Image
Examples
Embodiment Construction
[0052] Embodiments of the present invention will be described in detail below in conjunction with the accompanying drawings.
[0053] This embodiment includes the following steps:
[0054] S1. Prepare a face dataset, which includes face images and corresponding identity labels. The data set implemented in the present invention is the public WebFace face data set, which contains 10,575 celebrities and a total of about 490,000 face images. The WebFace face data has good diversity and is more suitable for training deep convolutional neural networks.
[0055] S2. Perform face detection and face key point detection on each image in the face data set, and obtain the position of the face key point in each image. This step can use any face detection method and face key point detection method. This example uses the Adaboost face detection method based on LBP features and the face key point detection method based on shape regression. The face key point method can detect 68 key points...
PUM
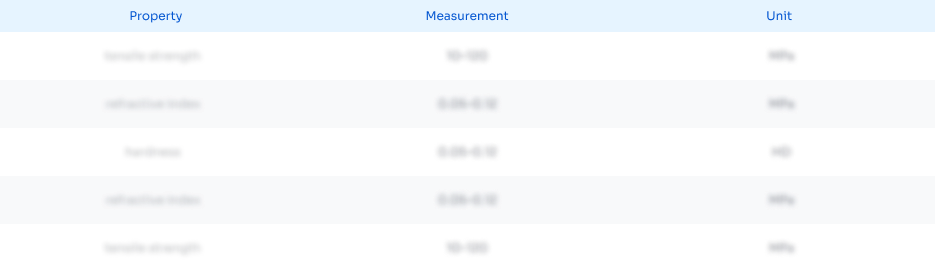
Abstract
Description
Claims
Application Information
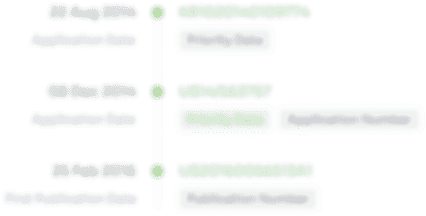
- Generate Ideas
- Intellectual Property
- Life Sciences
- Materials
- Tech Scout
- Unparalleled Data Quality
- Higher Quality Content
- 60% Fewer Hallucinations
Browse by: Latest US Patents, China's latest patents, Technical Efficacy Thesaurus, Application Domain, Technology Topic, Popular Technical Reports.
© 2025 PatSnap. All rights reserved.Legal|Privacy policy|Modern Slavery Act Transparency Statement|Sitemap|About US| Contact US: help@patsnap.com