Hyper-spectral image classification method based on singular value decomposition and neighborhood space information
A singular value decomposition and spatial information technology, applied in the field of hyperspectral image classification, can solve the problems of consuming a lot of time and money, having a large impact on classification results, and consuming a large amount of training time, so as to improve classification accuracy, improve automation level, shorten The effect of classification time
- Summary
- Abstract
- Description
- Claims
- Application Information
AI Technical Summary
Problems solved by technology
Method used
Examples
Embodiment Construction
[0015] The technical solutions and beneficial effects of the present invention will be described in detail below in conjunction with specific embodiments.
[0016] The present invention provides a hyperspectral image classification method based on singular value decomposition and neighborhood spatial information, comprising the following steps:
[0017] Step 1, input data: input the m×n training sample matrix of each class Among them, m is the number of training samples, n is the dimension of the hyperspectral vector, j represents its corresponding category (such as waters, roads, trees, etc.); at the same time, input the l×n test sample matrix D l×n , l is the number of test samples.
[0018] The following will enter the iterative loop to find the most suitable r value to get the best classification scheme.
[0019] Step 2, using formula A m×n =U m×r S r×r V r×n For the training sample matrix Perform singular value decomposition, where U m×r and V r×n are left and ...
PUM
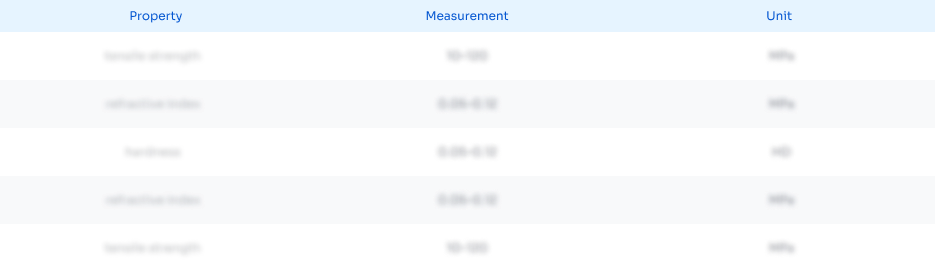
Abstract
Description
Claims
Application Information
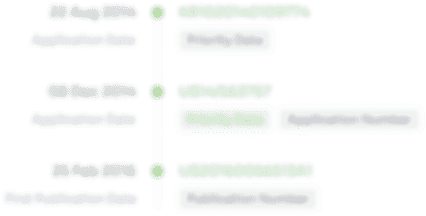
- R&D Engineer
- R&D Manager
- IP Professional
- Industry Leading Data Capabilities
- Powerful AI technology
- Patent DNA Extraction
Browse by: Latest US Patents, China's latest patents, Technical Efficacy Thesaurus, Application Domain, Technology Topic, Popular Technical Reports.
© 2024 PatSnap. All rights reserved.Legal|Privacy policy|Modern Slavery Act Transparency Statement|Sitemap|About US| Contact US: help@patsnap.com