Detecting system for Android malicious code based on deep learning and method thereof
A technology of malicious code detection and deep learning, applied in the field of Android malicious code detection system based on deep learning, can solve problems such as omission, roughness, and impact on classification accuracy, so as to simplify development, improve accuracy, and ensure accuracy Effect
- Summary
- Abstract
- Description
- Claims
- Application Information
AI Technical Summary
Problems solved by technology
Method used
Image
Examples
Embodiment Construction
[0065] Such as figure 1 As shown, the Android malicious code detection system based on deep learning of an embodiment of the present invention includes:
[0066] Feature extraction module: takes the APK program as input, uses a combination of static extraction and dynamic extraction, and outputs a vector composed of 0 and 1 as the feature vector of the APK program;
[0067] Deep Learning Module: Use a multi-layer perceptron (MLP) model as the learning model. On the one hand, the sample set composed of feature vectors and supervisory values is trained and learned to obtain a mature model; on the other hand, the feature vector is used as input, and the mature model is used to output the probability of the result as the security level of the APK program;
[0068] Report generation module: According to the feature vector and security level of the APK program, interpret and analyze, and generate an evaluation report.
[0069] The Android malicious code detection method based on...
PUM
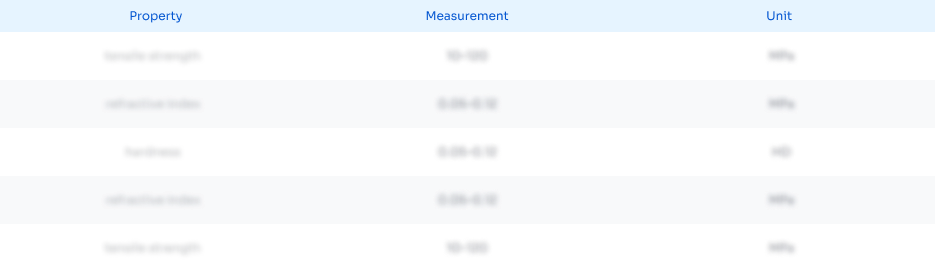
Abstract
Description
Claims
Application Information
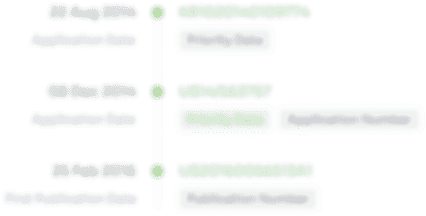
- Generate Ideas
- Intellectual Property
- Life Sciences
- Materials
- Tech Scout
- Unparalleled Data Quality
- Higher Quality Content
- 60% Fewer Hallucinations
Browse by: Latest US Patents, China's latest patents, Technical Efficacy Thesaurus, Application Domain, Technology Topic, Popular Technical Reports.
© 2025 PatSnap. All rights reserved.Legal|Privacy policy|Modern Slavery Act Transparency Statement|Sitemap|About US| Contact US: help@patsnap.com