Markov carpet embedded type feature selection method based on packaging
A feature selection method and embedded technology, applied in special data processing applications, instruments, electrical digital data processing, etc., can solve problems such as the inability to quickly identify redundant features
- Summary
- Abstract
- Description
- Claims
- Application Information
AI Technical Summary
Problems solved by technology
Method used
Image
Examples
Embodiment Construction
[0049] In this embodiment, it is assumed that the researched object is a data set Data composed of m instances, which is recorded as Data={inst 1 ,inst 2 ,...,inst i ,...,inst m}, for example, the data set Data can be microarray gene expression data; inst i Indicates the i-th instance; 1≤i≤m; the i-th instance inst i by n features i.e. the genes in the microarray data, and a categorical variable C i Composition, that is, the category corresponding to the microarray sample, such as cancer / normal; Indicates the i-th instance inst i The j-th feature in , 1≤j≤n; the j-th feature vector is composed of the j-th features of m instances, denoted as Thus, n feature vectors of m instances are obtained, denoted as f={f 1 , f 2 ,..., f j ,..., f n}; The category vector is composed of category variables of m instances, denoted as C={C 1 ,C 2 ,...,C i ,...,C m}; The attribute vector D of the data set Data is composed of n feature vectors f and category vector C var ={f 1...
PUM
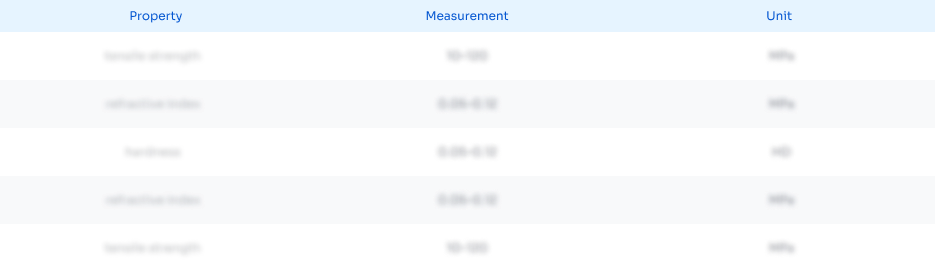
Abstract
Description
Claims
Application Information
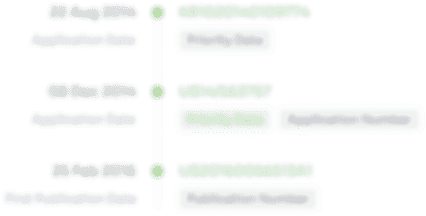
- Generate Ideas
- Intellectual Property
- Life Sciences
- Materials
- Tech Scout
- Unparalleled Data Quality
- Higher Quality Content
- 60% Fewer Hallucinations
Browse by: Latest US Patents, China's latest patents, Technical Efficacy Thesaurus, Application Domain, Technology Topic, Popular Technical Reports.
© 2025 PatSnap. All rights reserved.Legal|Privacy policy|Modern Slavery Act Transparency Statement|Sitemap|About US| Contact US: help@patsnap.com