Large-scale target identification method based on mobile platform
A target recognition and mobile platform technology, applied in the field of image recognition, can solve problems such as no longer applicable image retrieval
- Summary
- Abstract
- Description
- Claims
- Application Information
AI Technical Summary
Problems solved by technology
Method used
Image
Examples
Embodiment 1
[0106] Embodiment 1: First, some concepts in the description of the implementation steps are explained.
[0107] 1. Implementation steps of the training process
[0108] 1. First obtain the SIFT descriptor of each image in the image dataset.
[0109] 2. For the set of descriptors of all images in the data set, use the k-means iterative method to minimize the objective function: NS + MD. At the end of this process, we will have binary label information adapted to the distribution properties of the data points in Euclidean space.
[0110] 3. For datasets, divide the datasets into different groups according to their label information. Each group can get a weak hash function.
[0111] 4. Use the AdaBoost mechanism to combine weak hash functions into a strong hash function, further emphasizing that the resulting binary code can maintain the proximity between data points. The resulting binary code can approximately replace the Euclidean distance between data points. An inver...
Embodiment 2
[0126] Datasets: Two popular datasets are used as the retrieved datasets, namely the SIFT1M dataset and the CIFAR10 dataset. Among them, the SIFT1M data set contains a total of 1 million training data sets and 100,000 test data sets. While CIFAR10 contains 60,000 images. 50,000 images are used as the training set and 10,000 images are used as the test set. They all use Top-10as a measure of accuracy.
[0127] Evaluation index: use the general average retrieval accuracy ( mAP ), recall rate and average retrieval time to test the present invention and other best methods in the industry for comparison.
[0128] In the CIFAR10 data set, the feature descriptors of the training data set and the test data set are extracted through the SIFT algorithm. Under the two data sets respectively, use the present invention and the best methods in the industry (KMH[3], ITQ[4], RR[6], LSH[9], SH[5]) to retrieve the test data set respectively in the training nearest neighbors in the datas...
PUM
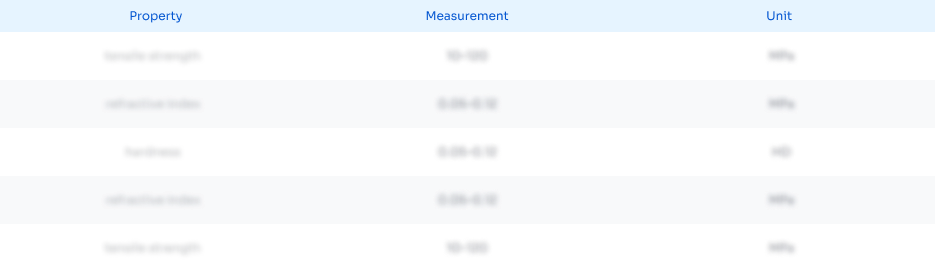
Abstract
Description
Claims
Application Information
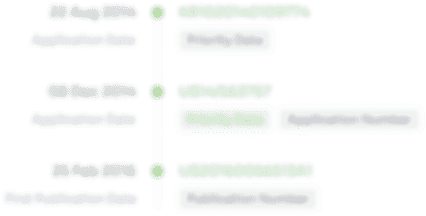
- R&D Engineer
- R&D Manager
- IP Professional
- Industry Leading Data Capabilities
- Powerful AI technology
- Patent DNA Extraction
Browse by: Latest US Patents, China's latest patents, Technical Efficacy Thesaurus, Application Domain, Technology Topic, Popular Technical Reports.
© 2024 PatSnap. All rights reserved.Legal|Privacy policy|Modern Slavery Act Transparency Statement|Sitemap|About US| Contact US: help@patsnap.com