PSO (Particle Swarm Optimization) extremity learning machine based strip steel exit thickness predicting method
An extreme learning machine and particle swarm optimization technology, applied in neural learning methods, prediction, biological neural network models, etc., can solve problems such as multi-hidden layer nodes and system ill-conditioning
- Summary
- Abstract
- Description
- Claims
- Application Information
AI Technical Summary
Problems solved by technology
Method used
Image
Examples
Embodiment Construction
[0044] One, theoretical basis of the present invention:
[0045] 1. The proposal of extreme learning machine
[0046] Extreme learning machine is a new type of single hidden layer feedforward neural network (SLFNs) learning algorithm, which was proposed by Huang Guangbin in 2004. In the extreme learning machine, the input weight connecting the input layer and the hidden layer and the bias value of the hidden layer are randomly selected, and the output weight connecting the hidden layer and the output layer is determined by the generalized inverse method analysis.
[0047] Given a training data set L={(x(n),t(n)),n=1,2,...,N}, where x(n)=(x 1 (n),...,x d (n)) T ∈ R d , t(n)=(t 1 (n),...,t m (n)) T ∈ R m . An extreme learning machine with activation function g( ) and H hidden layer neuron nodes can be expressed as formula (Ⅰ):
[0048] Σ j = 1 H β ...
PUM
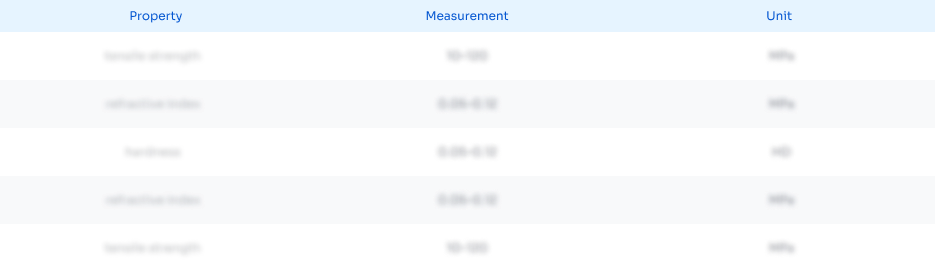
Abstract
Description
Claims
Application Information
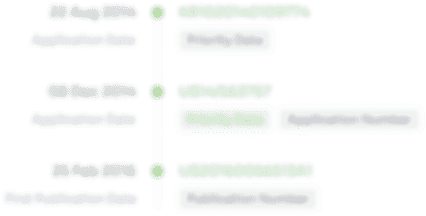
- R&D Engineer
- R&D Manager
- IP Professional
- Industry Leading Data Capabilities
- Powerful AI technology
- Patent DNA Extraction
Browse by: Latest US Patents, China's latest patents, Technical Efficacy Thesaurus, Application Domain, Technology Topic.
© 2024 PatSnap. All rights reserved.Legal|Privacy policy|Modern Slavery Act Transparency Statement|Sitemap