Sensor-fault diagnosing method based on online prediction of least-squares support-vector machine
A support vector machine and sensor failure technology, applied in the direction of instruments, computer components, character and pattern recognition, etc., can solve problems such as poor generalization ability, need, and large number of samples
- Summary
- Abstract
- Description
- Claims
- Application Information
AI Technical Summary
Problems solved by technology
Method used
Image
Examples
Embodiment Construction
[0026] The present invention provides a sensor fault diagnosis method based on least square support vector machine online prediction, its core idea is, as figure 1 As shown, the least squares support vector machine is selected to construct the online prediction model of the sensor. In the process of sensor sampling, a large window is used to slide in the measurement data to obtain the training data pool, and a small window is used to slide from the training data pool to obtain multiple groups. Training sample: use the sensor's rolling historical output data as a training sample to train the least squares support vector machine prediction model, and then when a new sample is input, the prediction model will predict the output value of the sensor at the next moment. By comparing the actual output of the sensor with the residual error generated by the least squares support vector machine prediction model output value, it is judged whether the fault occurs. If a fault is detected,...
PUM
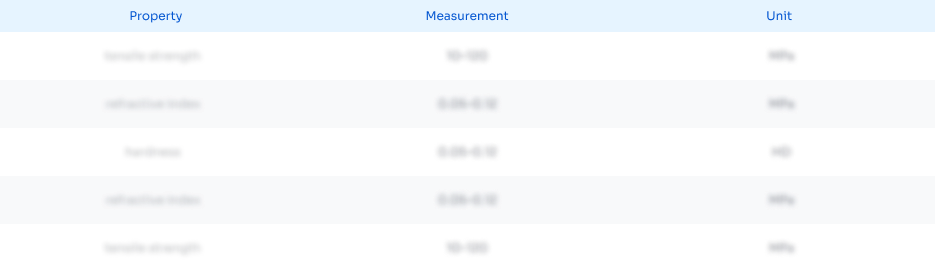
Abstract
Description
Claims
Application Information
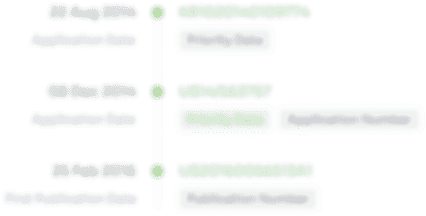
- R&D Engineer
- R&D Manager
- IP Professional
- Industry Leading Data Capabilities
- Powerful AI technology
- Patent DNA Extraction
Browse by: Latest US Patents, China's latest patents, Technical Efficacy Thesaurus, Application Domain, Technology Topic, Popular Technical Reports.
© 2024 PatSnap. All rights reserved.Legal|Privacy policy|Modern Slavery Act Transparency Statement|Sitemap|About US| Contact US: help@patsnap.com