Method for segmenting multi-dimensional texture image on basis of fuzzy C-means clustering and spatial information
A mean value clustering and texture image technology, applied in the field of image processing, can solve problems such as inaccurate segmentation edges and insufficient regional consistency, and achieve the effect of enhancing regional consistency, good regional consistency, and accurate edges
- Summary
- Abstract
- Description
- Claims
- Application Information
AI Technical Summary
Problems solved by technology
Method used
Image
Examples
Embodiment Construction
[0025] refer to figure 1 , the specific implementation process of the present invention is as follows:
[0026] Step 1, input the texture image to be segmented, the size of which is n×n, these texture images to be segmented can be synthetic texture images, or SAR images, aerial images and medical images with texture information.
[0027] Step 2, using the basis function DB7 to perform wavelet transformation on the input image, and the number of decomposition layers is four to obtain the wavelet coefficient w of the input image.
[0028] Step 3, calculating the eigenvectors corresponding to the wavelet coefficients at each transformation scale.
[0029] 3a) According to the Hidden Markov Tree HMT model, the corresponding relationship between the parent and child scales of the wavelet coefficients at each transformation scale is obtained, and this corresponding relationship is represented by a quadtree structure;
[0030] 3b) Approximating the wavelet coefficients using a Gaus...
PUM
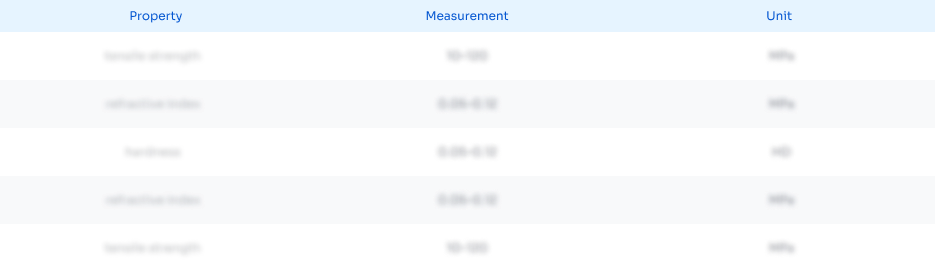
Abstract
Description
Claims
Application Information
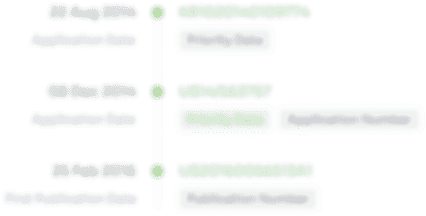
- R&D Engineer
- R&D Manager
- IP Professional
- Industry Leading Data Capabilities
- Powerful AI technology
- Patent DNA Extraction
Browse by: Latest US Patents, China's latest patents, Technical Efficacy Thesaurus, Application Domain, Technology Topic, Popular Technical Reports.
© 2024 PatSnap. All rights reserved.Legal|Privacy policy|Modern Slavery Act Transparency Statement|Sitemap|About US| Contact US: help@patsnap.com