Multi-classifier integrating method based on increment native Bayes network
A Bayesian network and multi-classifier technology, applied in the direction of instruments, calculations, electrical digital data processing, etc., can solve problems that affect classification prediction results, cannot discard useless classifiers in time, and concept interference, so as to avoid catastrophic forgetting , improve the effect of classification prediction results
- Summary
- Abstract
- Description
- Claims
- Application Information
AI Technical Summary
Problems solved by technology
Method used
Image
Examples
experiment example
[0060] The present invention adopts STAGGER, a classic data set of concept drift problem, to analyze the performance of DynamicAddExp (multi-classifier integration method based on incremental naive Bayesian network). The instance space of the STAGGER dataset is described by three attributes: size = {small, medium, large}, color = {red, green, blue}, and shape = {square, circular, triangular}. Class labels class ∈ {-1, +1}. Three target concepts are defined as follows: (1) size=small and color=red; (2) color=green or shape=circular; (3) size=(medium or large). 120 training instances are randomly generated, and each instance is assigned a category according to the current concept. Every 40 training examples belong to a concept, and the concept sequence is: (1)-(2)-(3). At each time step, the classifier learns from one instance and is tested for predictive accuracy on a test set of 100 instances. Test instances are also randomly generated according to the current concept. All...
PUM
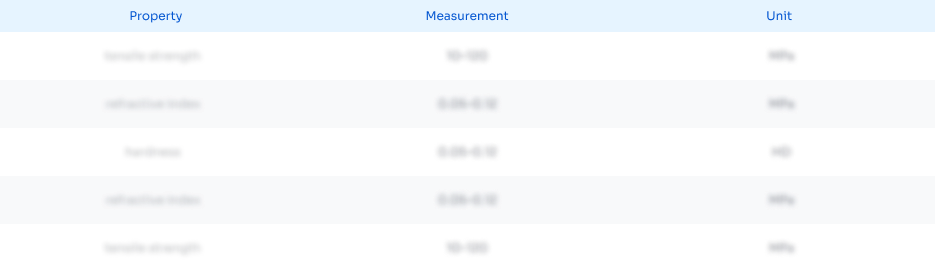
Abstract
Description
Claims
Application Information
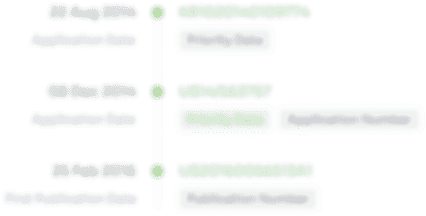
- R&D Engineer
- R&D Manager
- IP Professional
- Industry Leading Data Capabilities
- Powerful AI technology
- Patent DNA Extraction
Browse by: Latest US Patents, China's latest patents, Technical Efficacy Thesaurus, Application Domain, Technology Topic, Popular Technical Reports.
© 2024 PatSnap. All rights reserved.Legal|Privacy policy|Modern Slavery Act Transparency Statement|Sitemap|About US| Contact US: help@patsnap.com