Classification estimating system and classification estimating program
- Summary
- Abstract
- Description
- Claims
- Application Information
AI Technical Summary
Benefits of technology
Problems solved by technology
Method used
Image
Examples
embodiment 1
(Effect of Embodiment 1)
[0259]After attaching six EMG sensors SN1˜SN6 to the right wrist of a subject (refer to FIG. 1), said motion classification system S having said components start motion classification (refer to ST2 in FIG. 9) regarding to the seven motions (refer to FIG. 2) of a subject by selecting classification start button 1a (refer to FIG. 6) of motion classification start image 1.
[0260]Additionally, in said motion classification processing (refer to FIG. 9) of embodiment 1, the six surface EMG signals obtained from the six EMG sensors SNP˜SN6 are converted to the input value Ni(p) (p=1, 2, . . . , 6), Na(p), Nd(p) of the selective desensitization neutral network N (refer to FIG. 5) at each shift time, and are input into the input layer Na of said selective desensitization neutral network N (refer to ST4 of FIG. 9, ST101˜ST115 of FIG. 10). As a result, either −1 or +1 is input, at each shift time, into the thirty elements of the eight input element group Gi1(i=1, 2, . . ...
experimental examples
[0270]Here, in order to find out whether high classification accuracy with little training can be achieved by carrying out said input value generating processing as well as said motion classification processing, the following experimental example is prepared.
experimental example 1
[0271]In said motion classification system S of example 1, equivalent components of said motion classification system S of embodiment 1 are prepared, then said input value generating processing (refer to FIG. 10) as well as said motion classification processing (refer to FIG. 9) of embodiment 1 are carried out. Furthermore, said 60480 connection weights ωγijμ.ν as well as said 48 threshold values hiγ are determined when six motions are carried out twice respectively (two sets) on said subject at two second interval at three velocities of fast, medium, slow. That is to say, 36 (6×3×2) sample data are trained.
[0272]Besides, in said motion classification system S of example 1, six motions at three velocities, namely totally 18 motions are classified by carrying out six time (six sets) for actual motion classification.
[0273]In addition, in experimental example 1, Personal-EMG of Oisaka Electronic Device Ltd is applied as Surface EMG Measuring apparatus U1.
[0274]Moreover, in experimental...
PUM
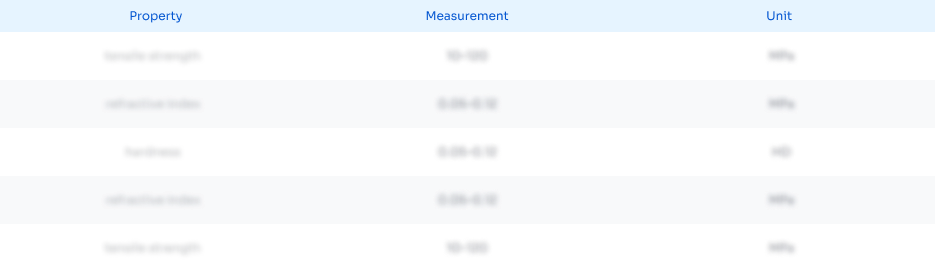
Abstract
Description
Claims
Application Information
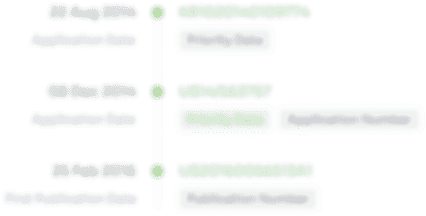
- R&D Engineer
- R&D Manager
- IP Professional
- Industry Leading Data Capabilities
- Powerful AI technology
- Patent DNA Extraction
Browse by: Latest US Patents, China's latest patents, Technical Efficacy Thesaurus, Application Domain, Technology Topic, Popular Technical Reports.
© 2024 PatSnap. All rights reserved.Legal|Privacy policy|Modern Slavery Act Transparency Statement|Sitemap|About US| Contact US: help@patsnap.com