Interpretable link prediction method for knowledge hypergraph
A prediction method and knowledge technology, applied in the field of knowledge hypergraph, to achieve the effect of improving performance and good link prediction
- Summary
- Abstract
- Description
- Claims
- Application Information
AI Technical Summary
Problems solved by technology
Method used
Image
Examples
Embodiment 1
[0047] The interpretable knowledge hypergraph constructed in the present invention represents the learning model, such as figure 1 As shown, an example graph of a knowledge hypergraph, related logic rules and a Markov blanket is given. Among them, the circle represents an entity, the ellipse in the upper figure represents several n-tuples of the knowledge hypergraph, the ellipse in the lower figure represents a group, and the n-tuple of the knowledge hypergraph consists of multiple entities, where parentsOf represents the parent-child relationship, and couple represents The relationship between husband and wife, wasBornIn represents the relationship of birthplace, and workAsFor represents the relationship of work status, which consists of 3, 2, 3, and 3 entities respectively. The rules based on these predicates are shown in the Markov logic network in the middle map. Markov A logical network consists of logical rules and rule weights. In the variational step E, the knowledge ...
Embodiment 2
[0049] Use the interpretable connection prediction method proposed by the present invention to realize the process of knowledge hypergraph representation learning, such as figure 2 As shown, the process of the knowledge hypergraph representation learning method is as follows:
[0050] 1. Establish an interpretable knowledge hypergraph representation learning model
[0051] 1-1) Input tuples and regular data, use Markov to build joint probabilities and maximize the log-likelihood of observable tuples.
[0052] Representing the knowledge hypergraph as in ε, and A finite set of knowledge hypergraphs, entities, relations, and observable tuples, respectively. Observable tuple t i =r(e 1 , e 2 ,...,e k ), where r is a relation, each is an entity, k is the non-negative integer number of relation r, and i is the observable tuple index. each tuple t i with an indicator variable association, indicates that the tuple is true, then indicates that the tuple is false...
Embodiment 3
[0093] The method of the present invention and the related methods in the prior art are the experimental results of link prediction on the knowledge hypergraph data set or knowledge map data set.
[0094] The present invention performs link prediction on knowledge hypergraph data sets JF17K, M-FB15K, FB-AUTO and knowledge graph data sets FB15k, WN18, FB15k-237, and WN18RR.
[0095] see image 3 , on the knowledge hypergraph dataset, m-CP is selected as the embedding model of the knowledge hypergraph. Compared with pure m-CP and other knowledge hypergraph embedding methods, the technical solution of the present invention achieves better performance on almost all evaluation indicators. The reason is that the knowledge hypergraph embedding method only utilizes the semantic information in the embedding space, while the present invention uses the embedding further combined with the domain knowledge in the logic rules to predict the establishment probability value of the hidden tup...
PUM
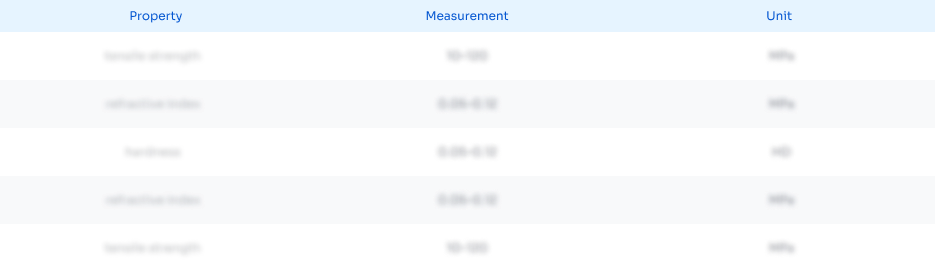
Abstract
Description
Claims
Application Information
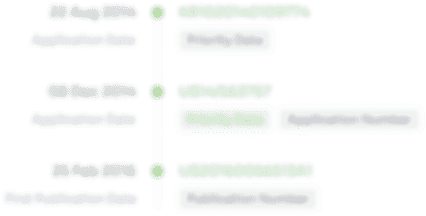
- R&D Engineer
- R&D Manager
- IP Professional
- Industry Leading Data Capabilities
- Powerful AI technology
- Patent DNA Extraction
Browse by: Latest US Patents, China's latest patents, Technical Efficacy Thesaurus, Application Domain, Technology Topic.
© 2024 PatSnap. All rights reserved.Legal|Privacy policy|Modern Slavery Act Transparency Statement|Sitemap