Incremental learning human body action recognition method based on CSI (Channel State Information)
A human action recognition and incremental learning technology, which is applied in the field of CSI-based incremental learning human action recognition, can solve problems such as the increase in the number of new action types, the decrease in the accuracy of action recognition, and the decrease in performance of old tasks, achieving recognition accuracy improvement, The effect of reducing the number of samples required and improving robustness
- Summary
- Abstract
- Description
- Claims
- Application Information
AI Technical Summary
Problems solved by technology
Method used
Image
Examples
Embodiment Construction
[0053] In this embodiment, figure 1 A flowchart for the implementation of incremental learning, such as figure 2 As shown, a CSI-based incremental learning human action recognition method is carried out as follows:
[0054] Step 1. Select M+1 indoor scenes with different layouts and record them as (C 0 ,C 1 ,…,C M ), where C 0 for the old scene, (C 1 ,…,C i ,…,C M ) is a new scene; C i Represents the i-th new scene; M represents the number of scenes;
[0055] A pair of WIFI transceivers are deployed in M+1 indoor scenarios respectively. Among them, the router is used as the sending device of the WIFI signal, denoted as AP, and the wireless network card is used as the receiving device, denoted as RP, and the router AP and wireless network card RP The separation distance is l; AP adopts TL-WDR6500 router, and RP adopts Intel 5300 network card;
[0056] Step 2. During the time period T, perform the s-th type of action on the collection point of any indoor scene, and us...
PUM
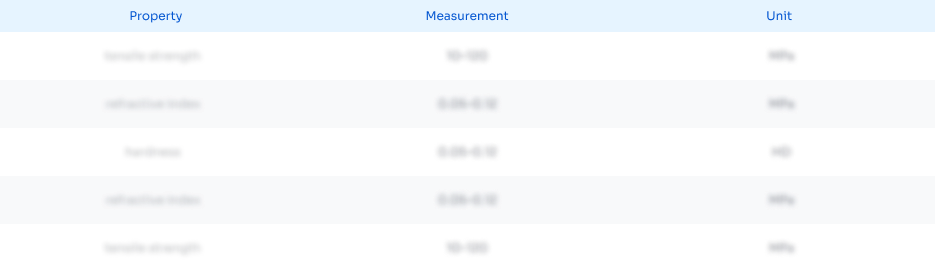
Abstract
Description
Claims
Application Information
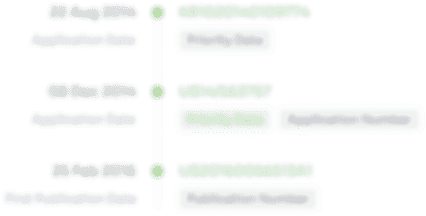
- Generate Ideas
- Intellectual Property
- Life Sciences
- Materials
- Tech Scout
- Unparalleled Data Quality
- Higher Quality Content
- 60% Fewer Hallucinations
Browse by: Latest US Patents, China's latest patents, Technical Efficacy Thesaurus, Application Domain, Technology Topic, Popular Technical Reports.
© 2025 PatSnap. All rights reserved.Legal|Privacy policy|Modern Slavery Act Transparency Statement|Sitemap|About US| Contact US: help@patsnap.com