Named entity recognition model training method, named entity recognition model application method and named entity recognition model training system
A named entity recognition and training method technology, applied in neural learning methods, character and pattern recognition, biological neural network models, etc., can solve the problems of low recognition accuracy and poor recognition effect
- Summary
- Abstract
- Description
- Claims
- Application Information
AI Technical Summary
Problems solved by technology
Method used
Image
Examples
Embodiment 1
[0072] Such as figure 1 As shown, the present embodiment provides a training method for a named entity recognition model, which specifically includes the following steps:
[0073] Step S1, preprocessing the fault text to obtain word vectors and word vectors; the word vectors include the word vectors of the named entity recognition task and the word vectors of the word segmentation task; the word vectors are used to judge two consecutive words in a sentence Whether the word vectors are related to the same word. Specifically include:
[0074] Step S1.1. Perform word segmentation processing on the fault text to obtain a word segmentation result. Specifically include:
[0075] Step S1.1.1. Segment the fault text data in units of sentences to obtain sentence segmentation results;
[0076] Step S1.1.2, segmenting the sentence segmentation result in units of words to obtain the word segmentation result;
[0077] Step S1.1.3, delete the stop words irrelevant to the named entity r...
Embodiment 2
[0178] Such as Figure 5 As shown, Embodiment 2 provides an application method of a named entity recognition model, which uses the trained named entity recognition model in Embodiment 1. It should be noted that the construction process and training process of the named entity recognition model will not be repeated in this embodiment. The specific process and calculation formula of each step are the same as those in Embodiment 1. Please refer to Embodiment 1, and neither repeat.
[0179] In this embodiment, the specific steps of the application method of the named entity recognition model are as follows:
[0180] Step T1, preprocessing the fault text to obtain word vectors; the word vectors include word vectors for named entity recognition tasks and word vectors for word segmentation tasks;
[0181] Step T2: Input the word vector of the named entity recognition task and the word vector of the word segmentation task into the trained named entity recognition model to obtain the...
Embodiment 3
[0187] This embodiment provides an application system for a named entity recognition model. When the application system is run by a processor, one or more steps in the training method for a named entity recognition model as described in Embodiment 1 are implemented, or, One or more steps in the application method of the named entity recognition model described in Embodiment 2.
PUM
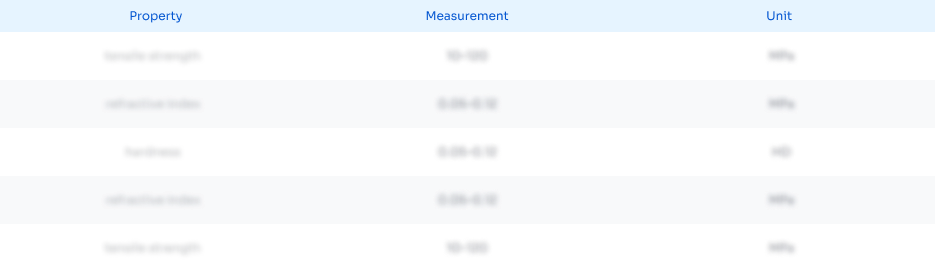
Abstract
Description
Claims
Application Information
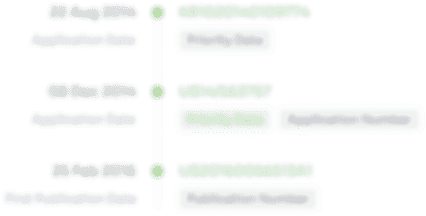
- R&D Engineer
- R&D Manager
- IP Professional
- Industry Leading Data Capabilities
- Powerful AI technology
- Patent DNA Extraction
Browse by: Latest US Patents, China's latest patents, Technical Efficacy Thesaurus, Application Domain, Technology Topic, Popular Technical Reports.
© 2024 PatSnap. All rights reserved.Legal|Privacy policy|Modern Slavery Act Transparency Statement|Sitemap|About US| Contact US: help@patsnap.com