Stock transaction method and system based on reinforcement learning algorithm and time sequence model
A technology of time series model and reinforcement learning, applied in computing model, machine learning, computing, etc., can solve the problems of uneven stock trend forecasting quality, difficulty for investors to obtain information value, difficulty in making trading decisions, etc.
- Summary
- Abstract
- Description
- Claims
- Application Information
AI Technical Summary
Problems solved by technology
Method used
Image
Examples
Embodiment
[0023] as attached figure 1 , 2 As shown, the stock trading method based on reinforcement learning algorithm and time series model in this embodiment includes the following steps:
[0024] S1. Data preprocessing
[0025] Sort the collected stock data by category, filter erroneous data and duplicate data, normalize the data, and obtain the stock data set after the preprocessing is completed.
[0026] S1.1. Data collection and arrangement
[0027] Organize the collected stock data by category into two main components: basic stock price data and enterprise operating index data. Among them, the basic stock price data include: opening price, closing price, highest price, lowest price, and trading volume during the stock trading period, and enterprise operating index data include: trading status, rolling price-earnings ratio, price-to-book ratio, rolling price-sales ratio, rolling market rate.
[0028] S1.2. Data filtering and cleaning
[0029] Filter and clean the collected o...
PUM
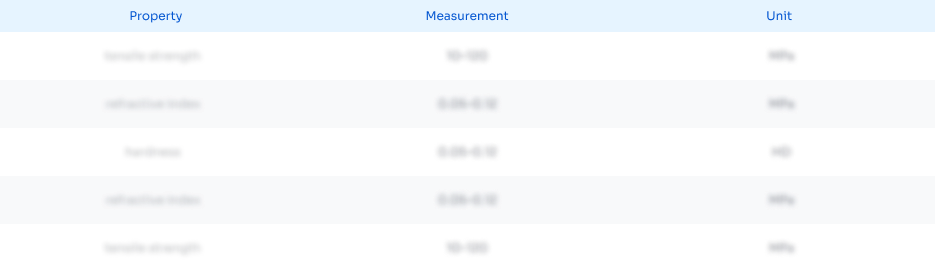
Abstract
Description
Claims
Application Information
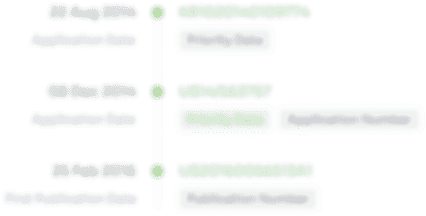
- R&D Engineer
- R&D Manager
- IP Professional
- Industry Leading Data Capabilities
- Powerful AI technology
- Patent DNA Extraction
Browse by: Latest US Patents, China's latest patents, Technical Efficacy Thesaurus, Application Domain, Technology Topic, Popular Technical Reports.
© 2024 PatSnap. All rights reserved.Legal|Privacy policy|Modern Slavery Act Transparency Statement|Sitemap|About US| Contact US: help@patsnap.com