Pedestrian trajectory prediction method based on graph partition convolutional neural network (GP-CNN)
A convolutional neural network and trajectory prediction technology, applied in the field of unmanned driving prediction planning, can solve problems such as direct learning, and achieve the effect of improving accuracy
- Summary
- Abstract
- Description
- Claims
- Application Information
AI Technical Summary
Problems solved by technology
Method used
Image
Examples
Embodiment
[0043] Step 1. Divide the input scene long trajectory data into multiple short trajectory data, and further divide the generated short trajectory into observation trajectory P obs and the true trajectory P pred . P obs After different preprocessing processes, it is converted into an embedded scene graph G=(V,E), and the scene graph G generates two input values of the graph division convolutional layer GP-CNN, which are respectively defined as observation trajectories And the Laplacian matrix A of each node.
[0044] The detailed description of the specific parameters of the embodiment is as follows:
[0045] The network structure of the graph division convolutional layer GP-CNN is as follows figure 1 , through a graph consisting of a convolutional layer for feature extraction, a pooling layer for downsampling, and a fully connected layer for weight classification to divide the channel and input scene trajectory data and the corresponding Laplacian matrix channel dual ...
PUM
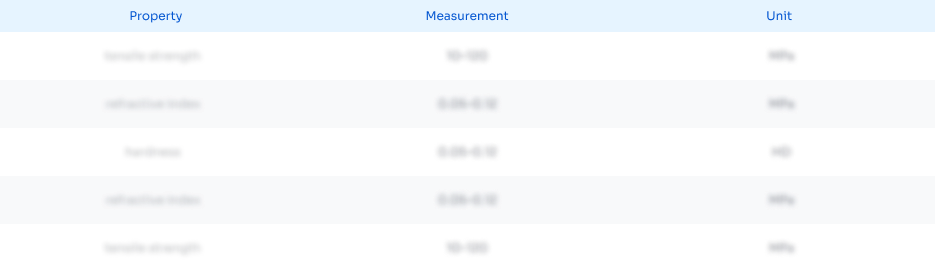
Abstract
Description
Claims
Application Information
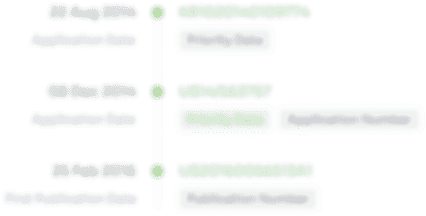
- R&D
- Intellectual Property
- Life Sciences
- Materials
- Tech Scout
- Unparalleled Data Quality
- Higher Quality Content
- 60% Fewer Hallucinations
Browse by: Latest US Patents, China's latest patents, Technical Efficacy Thesaurus, Application Domain, Technology Topic, Popular Technical Reports.
© 2025 PatSnap. All rights reserved.Legal|Privacy policy|Modern Slavery Act Transparency Statement|Sitemap|About US| Contact US: help@patsnap.com