Time sequence classification method based on LSTM and multi-scale FCN
A technology of time series and classification methods, applied in neural learning methods, character and pattern recognition, instruments, etc., can solve problems such as high attribute dimension, difficulty in solving global similarity, time step of data set sequence, etc., and achieve comprehensive beneficial information. Effect
- Summary
- Abstract
- Description
- Claims
- Application Information
AI Technical Summary
Problems solved by technology
Method used
Image
Examples
Embodiment Construction
[0036] The following will clearly and completely describe the technical solutions in the embodiments of the present invention with reference to the accompanying drawings in the embodiments of the present invention. Obviously, the described embodiments are only some, not all, embodiments of the present invention. Based on the embodiments of the present invention, other embodiments obtained by persons of ordinary skill in the art without making creative efforts all belong to the protection scope of the present invention.
[0037] Such as Figure 1-Figure 5b As shown, the present invention proposes a time series classification method based on LSTM and multi-scale FCN, taking dual-scale and three-scale convolution structures as specific examples, and the multi-scale FCN module and LSTM module that perform multi-scale convolution Composition, the multi-scale FCN module fully extracts the geometric space features of various granularities of the time series curve, the LSTM module is ...
PUM
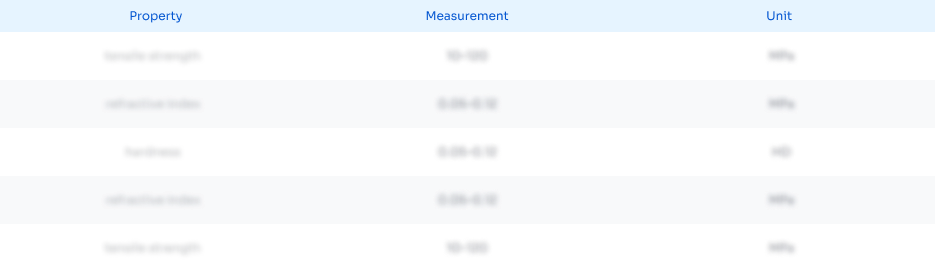
Abstract
Description
Claims
Application Information
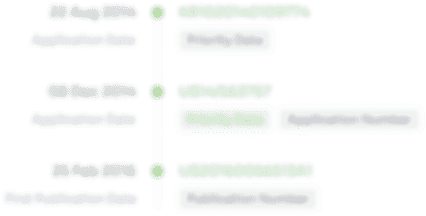
- Generate Ideas
- Intellectual Property
- Life Sciences
- Materials
- Tech Scout
- Unparalleled Data Quality
- Higher Quality Content
- 60% Fewer Hallucinations
Browse by: Latest US Patents, China's latest patents, Technical Efficacy Thesaurus, Application Domain, Technology Topic, Popular Technical Reports.
© 2025 PatSnap. All rights reserved.Legal|Privacy policy|Modern Slavery Act Transparency Statement|Sitemap|About US| Contact US: help@patsnap.com