Deep learning change detection method based on radar remote sensing data
A deep learning and change detection technology, applied in neural learning methods, instruments, biological neural network models, etc., can solve the problems of high cost of SAR image acquisition, few public data sets, insufficient research, etc., to avoid false changes, Avoid hard-to-obtain, avoid pseudo-change effects
- Summary
- Abstract
- Description
- Claims
- Application Information
AI Technical Summary
Problems solved by technology
Method used
Image
Examples
Embodiment Construction
[0034] The present invention will be further described in detail below in conjunction with the accompanying drawings and embodiments.
[0035] Step 1: The radar data used in this embodiment is the single-view complex (SLC, Single Look Complex) Radarsat-2 data of the fine beam mode full polarization (Fine Quad-Pol), and all the original radar data are according to the content of the invention step 1 The original image size is 2000×2000. The list of data details is shown in Table 1.
[0036] Table 1 Data Details
[0037]
[0038] Step 2: Build a development environment. The development environment of this embodiment is PyCharm, and the programming language is Python. The specific experimental hardware and software environment is shown in the following table.
[0039] Table 2 Details of hardware devices
[0040] hardware brand parameter graphics card NVIDIA GeForce RTX2080-TI(11GB) processor Intel Core I7-9700@3.60GHz8-core Memory Cors...
PUM
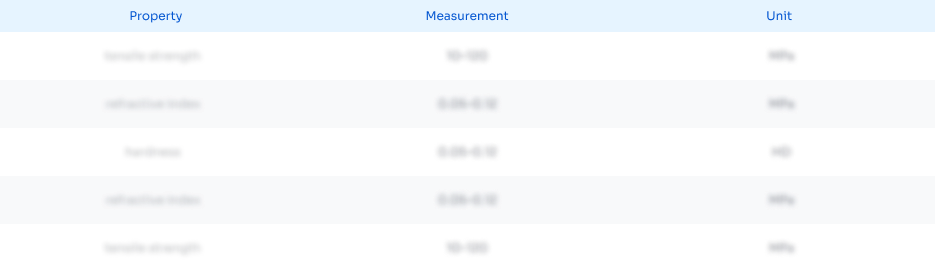
Abstract
Description
Claims
Application Information
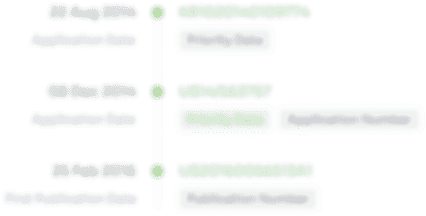
- R&D Engineer
- R&D Manager
- IP Professional
- Industry Leading Data Capabilities
- Powerful AI technology
- Patent DNA Extraction
Browse by: Latest US Patents, China's latest patents, Technical Efficacy Thesaurus, Application Domain, Technology Topic.
© 2024 PatSnap. All rights reserved.Legal|Privacy policy|Modern Slavery Act Transparency Statement|Sitemap