Optimized traffic flow prediction model based on space-time diagram convolutional network
A technology of convolutional network and predictive model, which is applied in traffic flow detection, biological neural network model, traffic control system of road vehicles, etc., can solve the problem of low performance, difficult analysis of traffic flow time-space dependence, and no consideration of prediction results Affect the size and other issues to achieve the effect of improving accuracy
- Summary
- Abstract
- Description
- Claims
- Application Information
AI Technical Summary
Problems solved by technology
Method used
Image
Examples
Embodiment Construction
[0053] The present invention will be further described in detail below in conjunction with the examples.
[0054] The overall structure of the model is as figure 1 shown. figure 1 Part (a) of the model represents the overall structure of the model, figure 1 Part (b) of ASTAGCN represents the specific details of the hidden layers of the model.
[0055] Definition of Traffic Flow Forecast:
[0056] Traffic flow prediction can be understood as predicting the traffic flow data of several time steps in the future based on the traffic flow data of several time steps recorded in the past for a specific road network structure, which can be expressed as equation (1).
[0057]
[0058] in, Represents the predicted value of the traffic flow data for several time steps in the future, which can be expressed as Equation (2). G=(V, E, A) represents the graph structure of the road network. V represents a collection of nodes in the graph, we regard each sensor that records traffic fl...
PUM
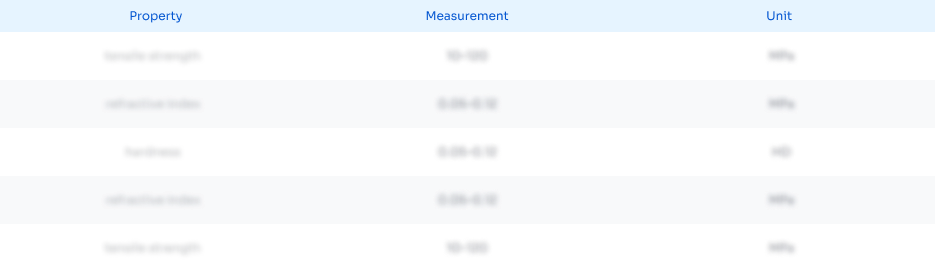
Abstract
Description
Claims
Application Information
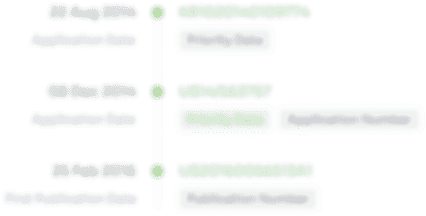
- Generate Ideas
- Intellectual Property
- Life Sciences
- Materials
- Tech Scout
- Unparalleled Data Quality
- Higher Quality Content
- 60% Fewer Hallucinations
Browse by: Latest US Patents, China's latest patents, Technical Efficacy Thesaurus, Application Domain, Technology Topic, Popular Technical Reports.
© 2025 PatSnap. All rights reserved.Legal|Privacy policy|Modern Slavery Act Transparency Statement|Sitemap|About US| Contact US: help@patsnap.com