Echo wall social network structure prediction method based on compressed sensing
A network structure and compressed sensing technology, applied in the field of text recognition, can solve problems such as lack of
- Summary
- Abstract
- Description
- Claims
- Application Information
AI Technical Summary
Problems solved by technology
Method used
Image
Examples
Embodiment 1
[0025] Step S1 HK model. The Hegselmann-Krause continuous opinion dynamics model (HK model) assumes that there are $n$ and two polarized opinions P and Q in the network, and opinions take values in a continuous interval. Each user i∈Rn has a continuous opinion xi, xi∈(0,1]. xi=0 means that user i supports opinion P, and xi=1 means that user i supports opinion Q. User x_i’s opinion is in [xi-ε, xi The interval of +ε]$ is affected by neighbors. The bounded confidence parameter ε is the key consensus threshold of the neighbors’ approach opinions that users intend to adopt. The discrete-time HK model can be described as
[0026]
[0027] Step S2 SBM model. A Stochastic Block Model (SBM) is used to generate the community structure. The generative network of SBM depends on the node connection probability given by the community relations. Nodes in the same community are more closely connected than nodes in another community. Considering the number k of communities, the prior...
PUM
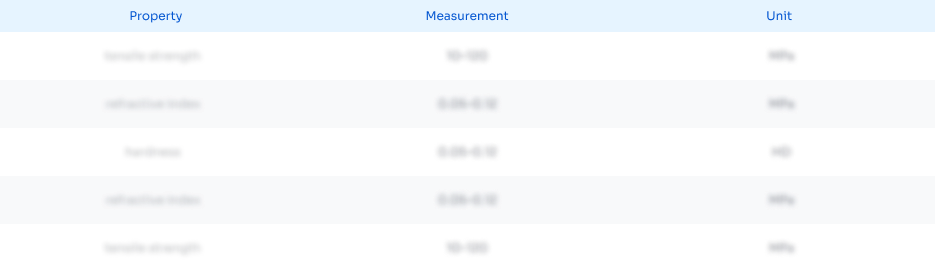
Abstract
Description
Claims
Application Information
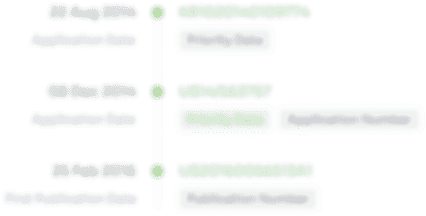
- R&D Engineer
- R&D Manager
- IP Professional
- Industry Leading Data Capabilities
- Powerful AI technology
- Patent DNA Extraction
Browse by: Latest US Patents, China's latest patents, Technical Efficacy Thesaurus, Application Domain, Technology Topic, Popular Technical Reports.
© 2024 PatSnap. All rights reserved.Legal|Privacy policy|Modern Slavery Act Transparency Statement|Sitemap|About US| Contact US: help@patsnap.com