Graph convolution-based relationship extraction method
A technology of relational extraction and convolution, applied in the field of relational extraction based on graph convolution, which can solve problems such as inaccurate features, lack of natural language processing tools, and method limitations
- Summary
- Abstract
- Description
- Claims
- Application Information
AI Technical Summary
Problems solved by technology
Method used
Image
Examples
Embodiment
[0065] Input data set: Enter the Linguistic Data Consortium official website to download the ACE 2005 data set. The data under the ACE2005 corpus folder includes Arabic, English and Chinese, and there are multiple data sources in each language.
[0066] With the help of natural language analysis tools, the original sentence in the data set is segmented to obtain the word segmentation result of the original sentence, so that the sentence after the word segmentation is obtained X =[ X 1 ,… X n ], using entity recognition tools to perform entity recognition on the original sentence, and the obtained entities are called subject entities and object entities according to the order in which they appear in the original sentence; with the help of natural language analysis tools, the original sentences in the data set are subjected to dependency syntax analysis , each word is represented as a node, and the semantic dependencies between words are used as the edges between the correspo...
PUM
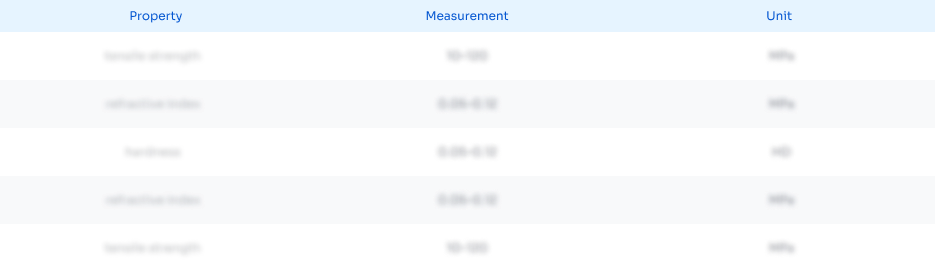
Abstract
Description
Claims
Application Information
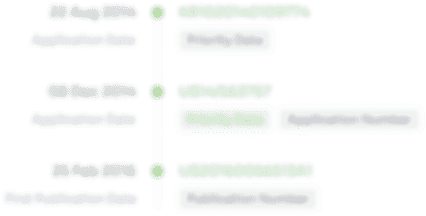
- R&D Engineer
- R&D Manager
- IP Professional
- Industry Leading Data Capabilities
- Powerful AI technology
- Patent DNA Extraction
Browse by: Latest US Patents, China's latest patents, Technical Efficacy Thesaurus, Application Domain, Technology Topic, Popular Technical Reports.
© 2024 PatSnap. All rights reserved.Legal|Privacy policy|Modern Slavery Act Transparency Statement|Sitemap|About US| Contact US: help@patsnap.com