Ultrahigh prediction method for track irregularity based on random oscillation sequence grey model
A track irregularity, grey model technology, applied in the field of track detection, can solve problems such as poor random oscillation sequence prediction effect, large error in random oscillation sequence prediction results, and inability to fit the sequence change trend.
- Summary
- Abstract
- Description
- Claims
- Application Information
AI Technical Summary
Problems solved by technology
Method used
Image
Examples
Embodiment 1
[0112] Such as figure 1 As shown, the present embodiment provides a method for superelevation prediction of track irregularity based on the random oscillation sequence gray model, the method comprising the following steps:
[0113] Data preprocessing step: performing mean value processing on the detected left and right rail surface height deviations to obtain an equidistant average height deviation sequence;
[0114] Preliminary prediction step: based on the gray model, the gray prediction of the random oscillation sequence is performed on the equidistant average height deviation sequence, and the preliminary prediction height deviation is obtained;
[0115] Prediction correction step: based on the preliminary forecast height deviation and the original data, the preliminary forecast height residual is obtained, the average height residual is calculated, and the preliminary forecast height residual is corrected based on the height residual average to obtain the corrected height...
Embodiment 2
[0204] This embodiment provides a superelevation prediction system based on a random oscillation sequence gray model, the system includes: a data preprocessing module, a preliminary prediction module, a prediction correction module, a neural network module and a superelevation prediction module;
[0205] Among them, the data preprocessing module is used to perform mean value processing on the detected left and right rail surface height deviations to obtain an equidistant average height deviation sequence;
[0206] The preliminary prediction module is used to carry out the gray prediction of the random oscillation sequence to the equidistant average height deviation sequence based on the gray model, and obtains the preliminary prediction height deviation;
[0207] The forecast correction module is used to obtain the preliminary forecast height residual based on the preliminary forecast height deviation and the original data, calculate the average height residual, and correct the...
PUM
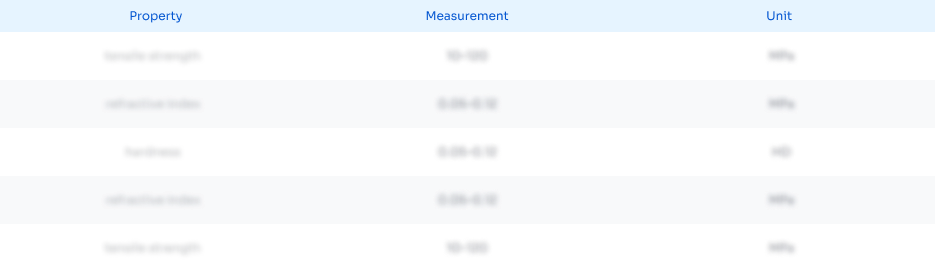
Abstract
Description
Claims
Application Information
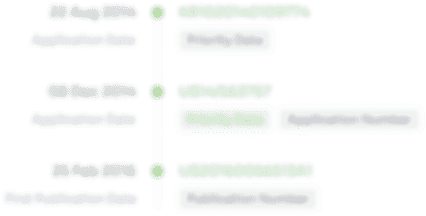
- R&D Engineer
- R&D Manager
- IP Professional
- Industry Leading Data Capabilities
- Powerful AI technology
- Patent DNA Extraction
Browse by: Latest US Patents, China's latest patents, Technical Efficacy Thesaurus, Application Domain, Technology Topic, Popular Technical Reports.
© 2024 PatSnap. All rights reserved.Legal|Privacy policy|Modern Slavery Act Transparency Statement|Sitemap|About US| Contact US: help@patsnap.com