Urban traffic accident risk prediction method based on a ConvLSTM
A long-short-term memory and traffic accident technology, applied in neural learning methods, biological neural network models, predictions, etc., can solve the problem of learning high-dimensional features and nonlinear relationships of multi-source heterogeneous data, and learning time-space sequence data at the same time Issues such as temporal correlation and spatial correlation
- Summary
- Abstract
- Description
- Claims
- Application Information
AI Technical Summary
Problems solved by technology
Method used
Image
Examples
Embodiment Construction
[0041] The present invention will be further described below in conjunction with the accompanying drawings.
[0042] refer to Figure 1 to Figure 4 , a traffic accident risk prediction method based on a recurrent neural network, comprising the following steps:
[0043] (1) Urban grid division: according to the geographic coordinates, the predicted city is divided into I×J grid areas of the same size, where each grid area is represented by an index (i, j);
[0044] (2) Construct the space-time risk matrix of traffic accidents: According to the time and place of traffic accidents, the accident risk of each area is counted at the same time period, and the risk matrix sequence of all grid areas changing with time is generated and normalized. Among them, the accident risk is the sum of the severity of each traffic accident in each region in each time period;
[0045] As an optimal solution, constructing the space-time risk matrix of traffic accidents is realized through the follo...
PUM
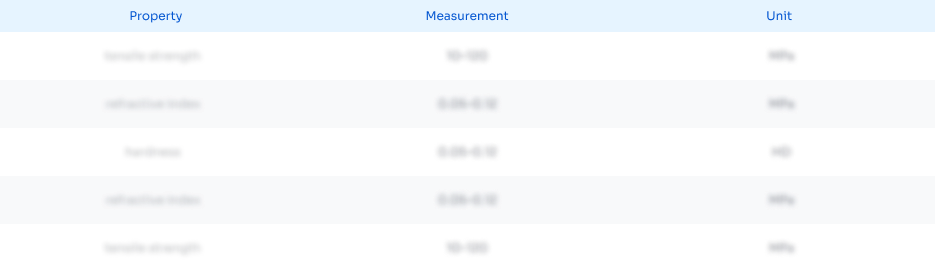
Abstract
Description
Claims
Application Information
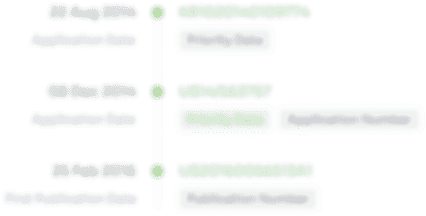
- R&D Engineer
- R&D Manager
- IP Professional
- Industry Leading Data Capabilities
- Powerful AI technology
- Patent DNA Extraction
Browse by: Latest US Patents, China's latest patents, Technical Efficacy Thesaurus, Application Domain, Technology Topic, Popular Technical Reports.
© 2024 PatSnap. All rights reserved.Legal|Privacy policy|Modern Slavery Act Transparency Statement|Sitemap|About US| Contact US: help@patsnap.com