A bidirectional cross-connected convolutional neural network for image segmentation
A convolutional neural network and image segmentation technology, applied in the field of convolutional neural network, can solve the problems of less skip connections, loss of texture information, unfavorable detection and integration of multi-level and multi-dimensional image information, and achieve high-precision results
- Summary
- Abstract
- Description
- Claims
- Application Information
AI Technical Summary
Problems solved by technology
Method used
Image
Examples
Embodiment Construction
[0020] The technical solutions in the embodiments of the present invention will be clearly and completely described below with reference to the accompanying drawings in the embodiments of the present invention. Obviously, the described embodiments are only a part of the embodiments of the present invention, rather than all the embodiments. Based on the embodiments of the present invention, all other embodiments obtained by those of ordinary skill in the art without creative efforts shall fall within the protection scope of the present invention.
[0021] refer to figure 1 , a bidirectional cross-connected convolutional neural network for image segmentation of the present invention, comprising the following steps:
[0022] Step 1, evaluate the advantages and disadvantages of the existing segmentation network (such as U-Net and BiO-Net), and build two different network branches on this basis to alleviate the problem of information loss caused by multiple image downsampling, and ...
PUM
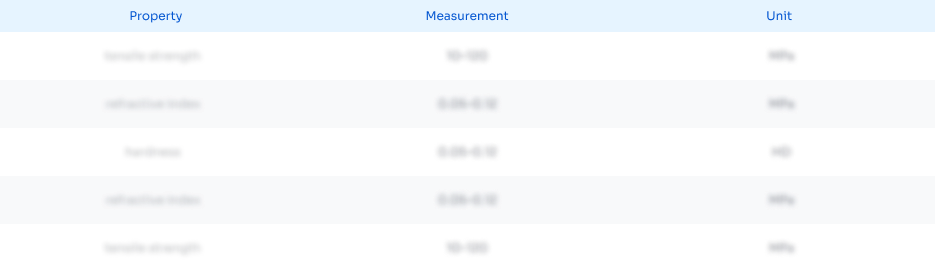
Abstract
Description
Claims
Application Information
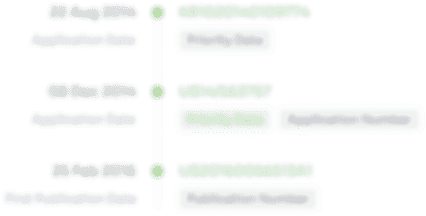
- R&D Engineer
- R&D Manager
- IP Professional
- Industry Leading Data Capabilities
- Powerful AI technology
- Patent DNA Extraction
Browse by: Latest US Patents, China's latest patents, Technical Efficacy Thesaurus, Application Domain, Technology Topic, Popular Technical Reports.
© 2024 PatSnap. All rights reserved.Legal|Privacy policy|Modern Slavery Act Transparency Statement|Sitemap|About US| Contact US: help@patsnap.com