A Discrete Asynchronous Event Data Augmentation Method
A technology of asynchronous events and event data, applied in the field of data processing, can solve the problem of low generalization ability of deep learning models, and achieve the effect of improving generalization ability, increasing diversity, and improving diversity
- Summary
- Abstract
- Description
- Claims
- Application Information
AI Technical Summary
Problems solved by technology
Method used
Image
Examples
Embodiment Construction
[0029] The preferred embodiments of the present invention will be described in detail below with reference to the accompanying drawings. It should be understood that the preferred embodiments are only for illustrating the present invention, rather than for limiting the protection scope of the present invention.
[0030] The present invention proposes three methods for enhancing event data, including random discarding, time discarding and regional discarding of a certain proportion of data. The random drop method is used to overcome the noise problem of event data, and the other two strategies are used to simulate different occlusion situations. figure 1 The idea of different event data augmentation strategies is illustrated. exist figure 1 where t represents the time dimension, x represents the pixel coordinates (only one dimension is shown here for clarity), the black dots represent the original events, the dots inside the rectangular dashed box represent the events to be...
PUM
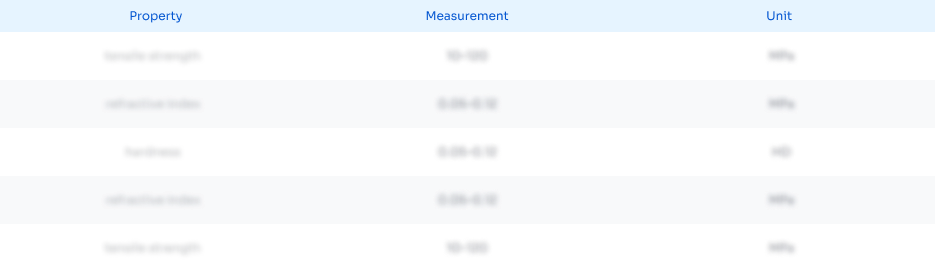
Abstract
Description
Claims
Application Information
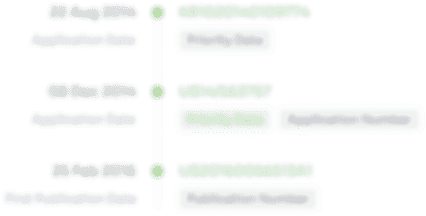
- Generate Ideas
- Intellectual Property
- Life Sciences
- Materials
- Tech Scout
- Unparalleled Data Quality
- Higher Quality Content
- 60% Fewer Hallucinations
Browse by: Latest US Patents, China's latest patents, Technical Efficacy Thesaurus, Application Domain, Technology Topic, Popular Technical Reports.
© 2025 PatSnap. All rights reserved.Legal|Privacy policy|Modern Slavery Act Transparency Statement|Sitemap|About US| Contact US: help@patsnap.com