A wavefront phase difference detection method based on long short-term memory deep network
A technology of long-term short-term memory and deep network, which is applied in the field of wavefront phase difference detection based on long-term short-term memory deep network. The effect of fast running speed, efficient algorithm, and simple algorithm
- Summary
- Abstract
- Description
- Claims
- Application Information
AI Technical Summary
Problems solved by technology
Method used
Image
Examples
Embodiment Construction
[0052] In order to make the technical solutions and advantages of the present invention more clear, the technical solutions in the embodiments of the present invention are clearly and completely described below in conjunction with the drawings in the embodiments of the present invention:
[0053] Such as figure 1 Shown is a wavefront phase difference detection method based on long-term short-term memory deep network. In the implementation process, the optical system uses the focus of the interferometer as a point light source. Get the PSF image respectively. The interferometer introduces the phase difference of the optical system by slightly tilting or translating the lens, and can directly measure the distortion phase difference of the optical system. The concrete steps of the method disclosed by the invention are as follows:
[0054] S1: Input the parameter information of the optical system: wavelength 0.6328μm, aperture size 8.5mm, focal length 180mm, detector pixel size ...
PUM
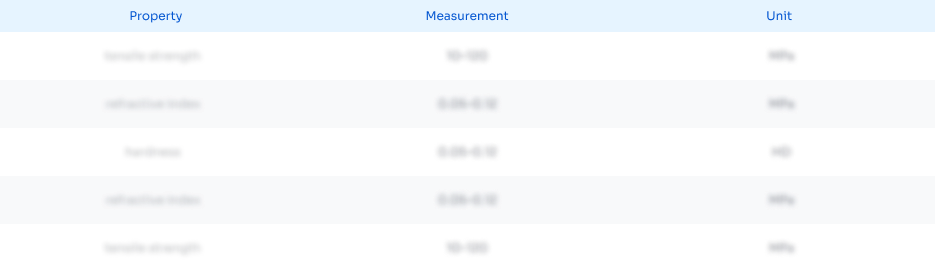
Abstract
Description
Claims
Application Information
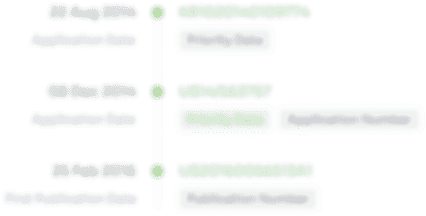
- R&D Engineer
- R&D Manager
- IP Professional
- Industry Leading Data Capabilities
- Powerful AI technology
- Patent DNA Extraction
Browse by: Latest US Patents, China's latest patents, Technical Efficacy Thesaurus, Application Domain, Technology Topic.
© 2024 PatSnap. All rights reserved.Legal|Privacy policy|Modern Slavery Act Transparency Statement|Sitemap