Multi-aircraft cooperative air combat planning method and system based on deep reinforcement learning
A reinforcement learning and planning technology, applied in neural learning methods, stochastic CAD, design optimization/simulation, etc., can solve problems such as difficulty in solving, large amount of calculation, inability to meet real-time decision-making, etc., to achieve good training effect and improve exploration ability. Effect
- Summary
- Abstract
- Description
- Claims
- Application Information
AI Technical Summary
Problems solved by technology
Method used
Image
Examples
Embodiment Construction
[0049] The technical solutions of the present invention will be clearly and completely described below in conjunction with the accompanying drawings. Apparently, the described embodiments are some of the embodiments of the present invention, but not all of them. Based on the embodiments of the present invention, all other embodiments obtained by persons of ordinary skill in the art without making creative efforts belong to the protection scope of the present invention.
[0050] Such as Figure 4 The specific battlefield situation information map of the experimental scenario environment is shown. In this scenario, the red and blue forces are configured equally, each containing 3 fighter jets and a base that can take off and land aircraft. The scope of the scenario is a rectangular high sea with a length of 1400 kilometers and a width of 1000 kilometers area. The process of the scenario deduction is that the aircraft takes off from the base, escorts the own base, and destroys t...
PUM
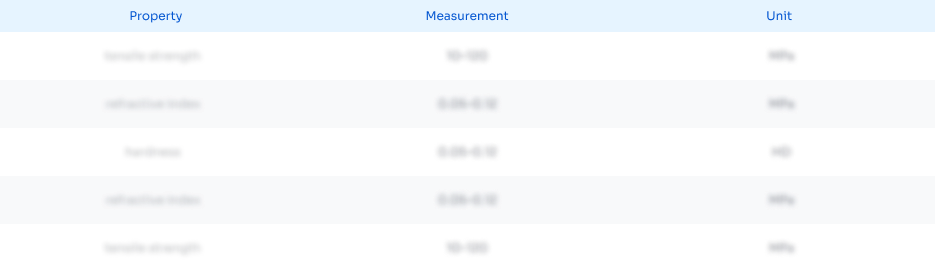
Abstract
Description
Claims
Application Information
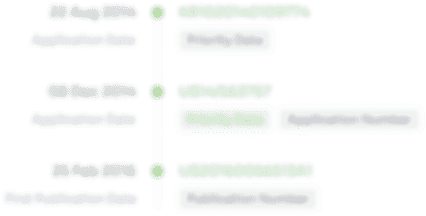
- R&D Engineer
- R&D Manager
- IP Professional
- Industry Leading Data Capabilities
- Powerful AI technology
- Patent DNA Extraction
Browse by: Latest US Patents, China's latest patents, Technical Efficacy Thesaurus, Application Domain, Technology Topic, Popular Technical Reports.
© 2024 PatSnap. All rights reserved.Legal|Privacy policy|Modern Slavery Act Transparency Statement|Sitemap|About US| Contact US: help@patsnap.com