Traffic prediction method based on adaptive spatial self-attention map convolution
A technology of traffic prediction and attention, applied in the field of transportation and deep learning, can solve problems such as the inability to model global spatial correlation, complex spatio-temporal correlation, and the inability to capture dynamic changes in road network structure
- Summary
- Abstract
- Description
- Claims
- Application Information
AI Technical Summary
Problems solved by technology
Method used
Image
Examples
Embodiment Construction
[0102]The model saved during the loading training process is obtained according to step 1) -4 in the training process, and then the prediction result is reversed:
[0103]
[0104]among them,Is a forecast result, σxAnd M represent the sample standard difference and the average value, the same as the formula (1),Indicates the result of the inverselation. Then calculate the average absolute error MAE, the root mean square error RMSE and the average absolute percentage error MAPE these three performance indicators, the three performance indicators are defined as follows:
[0105]
[0106]
[0107]
[0108]Where Xi,The first elements in the real value and the predicted value are respectively, and n represents the total number of elements.
[0109]We use the 1-hour historical data to predict the traffic flow in the next 1 hour, compared with the three models of STGCN, ASTGCN, DCRNN, and the experimental results on the two data sets are shown in the following table:
[0110]Table 1 Comparison of STGCN, ASTGCN, D...
PUM
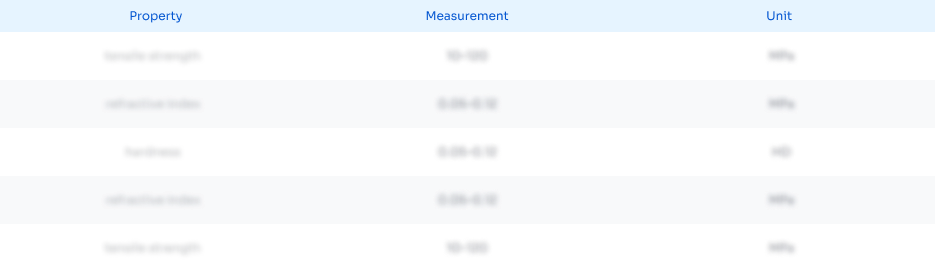
Abstract
Description
Claims
Application Information
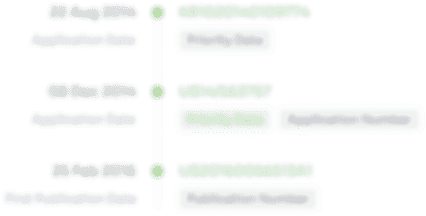
- R&D Engineer
- R&D Manager
- IP Professional
- Industry Leading Data Capabilities
- Powerful AI technology
- Patent DNA Extraction
Browse by: Latest US Patents, China's latest patents, Technical Efficacy Thesaurus, Application Domain, Technology Topic, Popular Technical Reports.
© 2024 PatSnap. All rights reserved.Legal|Privacy policy|Modern Slavery Act Transparency Statement|Sitemap|About US| Contact US: help@patsnap.com