Multi-attribute target classification method based on sample set
A classification method, a technique for sample sets, applied in the fields of instruments, character and pattern recognition, computer parts, etc.
- Summary
- Abstract
- Description
- Claims
- Application Information
AI Technical Summary
Problems solved by technology
Method used
Image
Examples
Embodiment 1
[0052] Example 1: Basic Classification Method
[0053]The process flow of the classification method of the multi-attribute target based on the sample set in the present invention is as follows: Figure 6 shown. by figure 1 Take the known sample set shown as an example. There are two types of samples in the known sample set, one is triangular and the other is five-star. Each sample has two attributes, A and B, and the category is a subset of five-star samples. The value range of the A attribute is [2,9], the value range of the B attribute is [5,9]; the value range of the A attribute of the sample subset whose category is triangle is [3,10], and the B attribute The value range of is [1,6]. From the perspective of the entire sample set, the value range of attribute A is [2,10], and the value span is 10-2=8; the value range of attribute B is [1,9], and the value span is 9-1 =8.
[0054] Targets to be classified such as figure 2 As shown in the circle, the purpose of classif...
Embodiment 2
[0058] Example 2: The strategy for the order of attribute selection in the sample set screening process
[0059] In the first embodiment, the difficulty of obtaining the values of target attribute A and attribute B is not considered, so it is not important to filter from attribute A first or filter from attribute B first. However, the cost of obtaining different attributes of the actual target is different. For example, for a cup, the color of the cup is easy to obtain, while the density of the cup is more difficult to obtain. In the classification process, if we can directly implement classification from an easily obtained attribute, there is no need to obtain hard-to-obtain attributes and reduce the cost of classification.
[0060] That is to say, in the process of traversing attributes to filter sample sets, it is a more cost-effective classification method to preferentially filter from the threshold range of attributes with lower cost.
Embodiment 3
[0061] Example 3: The selected sample subset is empty, and the target to be classified is manually classified and added to the sample set
[0062] Such as Figure 5 As shown, the value of attribute A of the target to be classified is 12, and the value of attribute B is 11. The screening threshold range of attribute A is [11,13], and the screening threshold range of attribute B is [10,12]. From the figure It can be seen that no matter which attribute is used to filter, the obtained sample subset is empty.
[0063] At this time, the target to be classified can be submitted to manual classification. After the target category is manually determined, the target is added as a sample to the sample set as a sample set to be screened. If there are targets with similar values that need to be classified later, you can pass the aforementioned classification. method to get the target category.
PUM
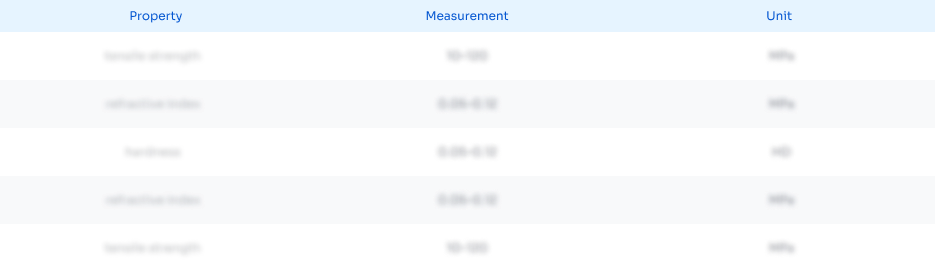
Abstract
Description
Claims
Application Information
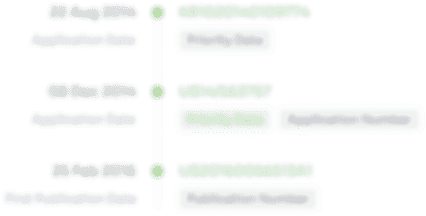
- R&D Engineer
- R&D Manager
- IP Professional
- Industry Leading Data Capabilities
- Powerful AI technology
- Patent DNA Extraction
Browse by: Latest US Patents, China's latest patents, Technical Efficacy Thesaurus, Application Domain, Technology Topic, Popular Technical Reports.
© 2024 PatSnap. All rights reserved.Legal|Privacy policy|Modern Slavery Act Transparency Statement|Sitemap|About US| Contact US: help@patsnap.com