Small target detection method based on improved Faster R-CNN
A small target detection and algorithm technology, applied in the field of target detection, can solve the problems of false detection and missed detection, low efficiency, high labor intensity, etc., and achieve high recognition accuracy, good recognition and detection effect
- Summary
- Abstract
- Description
- Claims
- Application Information
AI Technical Summary
Problems solved by technology
Method used
Image
Examples
Embodiment Construction
[0032] The present invention will be further described below in conjunction with the accompanying drawings.
[0033] First of all, the process of feature extraction of cloth defect images using the VGG16 network model is as follows: image 3 shown. VGG16 contains a total of 13 convolutional layers and 5 pooling layers. The convolution operation will not change the size of the feature map transmitted by the previous layer, and the step size of each pooling layer is 2, and the size of the feature map will be reduced to half after pooling. According to the collected cloth samples, the length of the input image is 2446, the width is 1000, and the number of channels is 3. The feature extraction is performed through the VGG16 network. The final output feature map has a length of 76, a width of 31, and the number of channels. for 512.
[0034] In the convolutional network that generates feature maps, the neurons that generate the underlying feature maps have less pre-computation s...
PUM
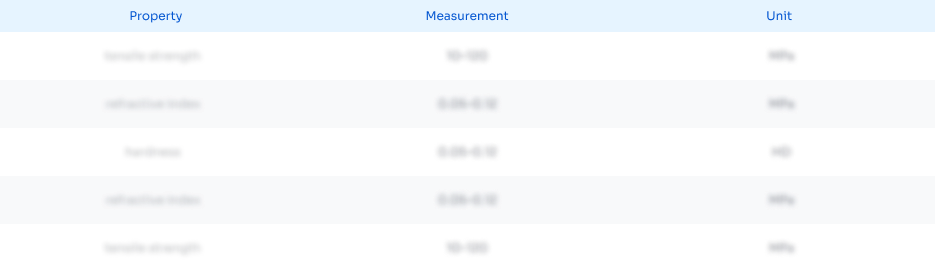
Abstract
Description
Claims
Application Information
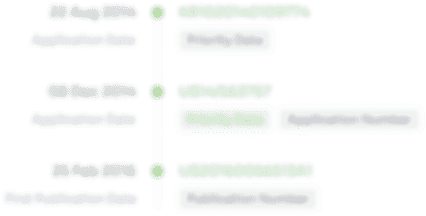
- Generate Ideas
- Intellectual Property
- Life Sciences
- Materials
- Tech Scout
- Unparalleled Data Quality
- Higher Quality Content
- 60% Fewer Hallucinations
Browse by: Latest US Patents, China's latest patents, Technical Efficacy Thesaurus, Application Domain, Technology Topic, Popular Technical Reports.
© 2025 PatSnap. All rights reserved.Legal|Privacy policy|Modern Slavery Act Transparency Statement|Sitemap|About US| Contact US: help@patsnap.com