Power load prediction method based on phase space reconstruction and data driving
A phase space reconstruction and power load technology, which is applied in forecasting, kernel methods, data processing applications, etc., can solve the problem of low forecasting accuracy and achieve the effect of improving accuracy
- Summary
- Abstract
- Description
- Claims
- Application Information
AI Technical Summary
Problems solved by technology
Method used
Image
Examples
Embodiment 1
[0064] See Figure 1 to Figure 10 , an embodiment of the present invention provides a phase space reconstruction and data-driven power load forecasting method, including:
[0065] S1: Calculate the delay time and embedding dimension of the historical load data, divide the historical load data into a training set and a test set, reconstruct the phase space of the training set and the test set according to the delay time and embedding dimension, and perform maximum and minimum normalized processing;
[0066] S2: Input the training set and test set after the maximum and minimum normalization processing into at least two types of data-driven models, wherein each type of data-driven model contains one or more data-driven models for autonomous learning input Data characteristics of the data set and output prediction results, compare the prediction accuracy of all data-driven models, and use preset evaluation indicators to select the optimal model corresponding to different types of...
PUM
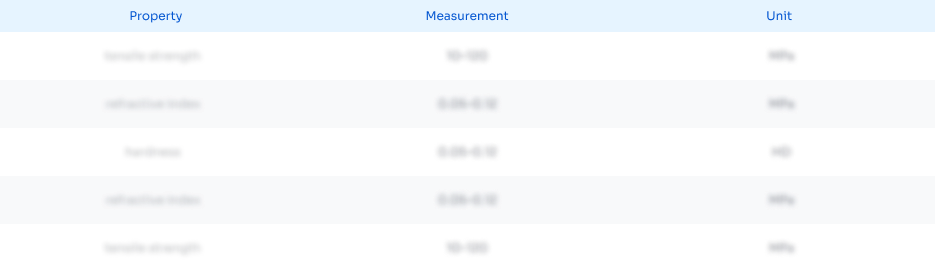
Abstract
Description
Claims
Application Information
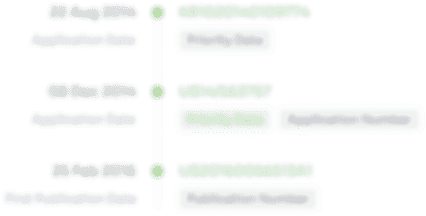
- Generate Ideas
- Intellectual Property
- Life Sciences
- Materials
- Tech Scout
- Unparalleled Data Quality
- Higher Quality Content
- 60% Fewer Hallucinations
Browse by: Latest US Patents, China's latest patents, Technical Efficacy Thesaurus, Application Domain, Technology Topic, Popular Technical Reports.
© 2025 PatSnap. All rights reserved.Legal|Privacy policy|Modern Slavery Act Transparency Statement|Sitemap|About US| Contact US: help@patsnap.com