Multi-agent reinforcement learning method and system based on hierarchical attention mechanism
A multi-agent and reinforcement learning technology, applied in neural learning methods, neural architectures, biological neural network models, etc., can solve problems such as unscalability, increase in the size of state space and action space, and difficulty in realizing communication requirements, etc., to achieve The effect of improving scalability
- Summary
- Abstract
- Description
- Claims
- Application Information
AI Technical Summary
Problems solved by technology
Method used
Image
Examples
Embodiment 1
[0062] combine figure 1 , the present invention provides a multi-agent reinforcement learning method based on hierarchical attention mechanism, comprising:
[0063] Build a learning environment that includes multiple agents;
[0064] The critic network calculates the estimated value based on the observation and action values of other agents in multiple agents obtained by the hierarchical attention mechanism of each agent, and optimizes it through the minimum joint loss function until the minimum joint loss function converges;
[0065] Based on the observation value, action value and estimated value after training, the action-value function is calculated by estimating the Actor network and optimized by maximizing the advantage function until the optimal action-value function is obtained;
[0066] Deterministic actions are performed based on an optimal action-value function.
[0067] Build a learning environment that includes multiple agents, including:
[0068] Based on th...
Embodiment 2
[0103] combine figure 2 , the present invention comprises the following steps:
[0104] The first step, build the combination image 3intensive learning environment. It mainly includes three kinds of cooperation environment and hunting environment (mixed environment). The environment simulates a real physical world environment, where there are elastic forces, resistance, etc. Specific steps are as follows:
[0105] 1.1 Collaborative navigation scenario: In this scenario, X agents try to reach L preset target points (L=X) through cooperation. All agents only have physical actions, but can observe their relative positions with other agents and target points. The reward feedback of the agent is related to the distance from each target to any agent, so it is required that the agent must cover each target in order to obtain the maximum reward. And, when the agent collides with other agents, it will get a penalty. We try to let the agent learn to cover all objects and avoid ...
Embodiment 3
[0172] Learning environment module: build a learning environment, which includes multiple agents;
[0173] Critic module: The critic network calculates the estimated value based on the observation values and action values of other agents in multiple agents obtained by the hierarchical attention mechanism of each agent, and optimizes through the minimum joint loss function until the minimum joint loss function convergence;
[0174] Actor network module: Based on the observation value, action value and estimated value after training, the estimated Actor network calculates the action-value function and optimizes it by maximizing the advantage function until the optimal action-value function is obtained;
[0175] Execution Action Module: Based on the optimal action-value function, execute deterministic actions.
[0176] The acquisition module includes an estimated value submodule, a minimum joint loss function submodule and an optimization submodule;
[0177] The Critic modu...
PUM
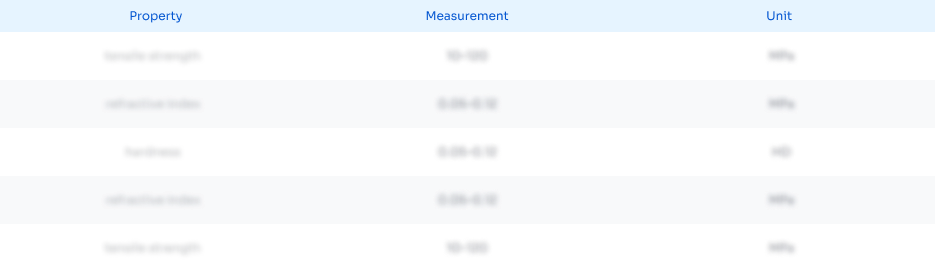
Abstract
Description
Claims
Application Information
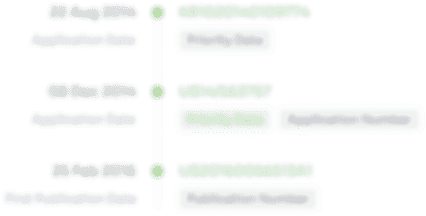
- R&D Engineer
- R&D Manager
- IP Professional
- Industry Leading Data Capabilities
- Powerful AI technology
- Patent DNA Extraction
Browse by: Latest US Patents, China's latest patents, Technical Efficacy Thesaurus, Application Domain, Technology Topic, Popular Technical Reports.
© 2024 PatSnap. All rights reserved.Legal|Privacy policy|Modern Slavery Act Transparency Statement|Sitemap|About US| Contact US: help@patsnap.com