PM2.5 high-precision space-time prediction method based on deep learning
A deep learning and high-precision technology, applied in the field of artificial intelligence and information applications, can solve the problems of small number of monitoring sites, sparse and uneven distribution, and no time-dependent feature integration, etc., to achieve the effect of improving accuracy
- Summary
- Abstract
- Description
- Claims
- Application Information
AI Technical Summary
Problems solved by technology
Method used
Image
Examples
Embodiment Construction
[0033] In order to facilitate those of ordinary skill in the art to understand and implement the present invention, the present invention will be described in further detail below in conjunction with the accompanying drawings and embodiments. It should be understood that the implementation examples described here are only used to illustrate and explain the present invention, and are not intended to limit this invention.
[0034] as attached figure 1 As shown, the PM based on deep learning provided by the present invention 2.5 A method for spatial-temporal prediction of concentration, comprising the following steps:
[0035] Step 1: PM on the ground monitoring site 2.5 Data, meteorological data, spatial correlation data and physical characteristic data are preprocessed. By collecting multi-source data and analyzing influencing factors, spatial correlation data and physical characteristic data are selected as relevant auxiliary data, and data preprocessing includes eliminatin...
PUM
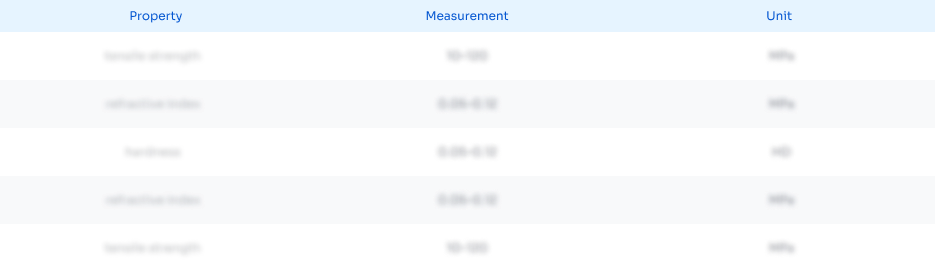
Abstract
Description
Claims
Application Information
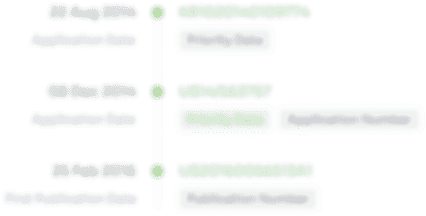
- R&D Engineer
- R&D Manager
- IP Professional
- Industry Leading Data Capabilities
- Powerful AI technology
- Patent DNA Extraction
Browse by: Latest US Patents, China's latest patents, Technical Efficacy Thesaurus, Application Domain, Technology Topic, Popular Technical Reports.
© 2024 PatSnap. All rights reserved.Legal|Privacy policy|Modern Slavery Act Transparency Statement|Sitemap|About US| Contact US: help@patsnap.com