A Generative Zero-Sample Prediction Method Based on Knowledge Graph
A technology of knowledge graph and sample prediction, applied in the field of generative zero-sample learning, which can solve problems such as difficulty in extracting useful information and high noise.
- Summary
- Abstract
- Description
- Claims
- Application Information
AI Technical Summary
Problems solved by technology
Method used
Image
Examples
Embodiment Construction
[0031] In order to make the objectives, technical solutions and advantages of the present invention clearer, the present invention will be further described in detail below with reference to the accompanying drawings and embodiments. It should be understood that the specific embodiments described herein are only used to explain the present invention, and do not limit the protection scope of the present invention.
[0032]The generative zero-sample prediction method based on the knowledge map provided by the present invention can be used in but not limited to the application scenarios where new categories appear in the fields of image classification, text classification, relationship classification and the like and lack of training samples. Rich semantic information solves the problem of learning and predicting zero-sample categories. This embodiment takes zero-sample animal image classification as an example, and tests the classification performance of images in zero-sample sce...
PUM
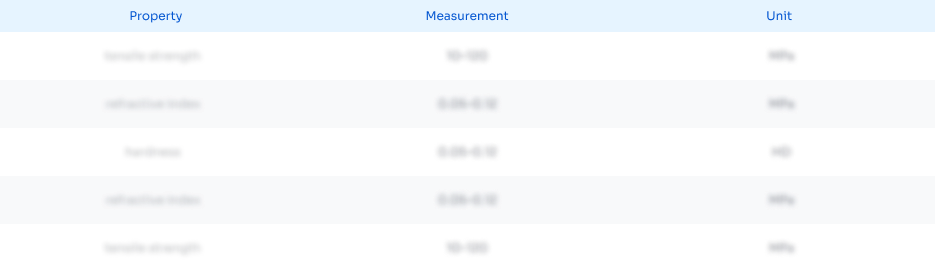
Abstract
Description
Claims
Application Information
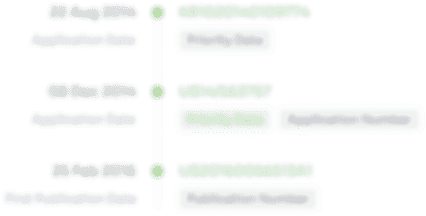
- R&D Engineer
- R&D Manager
- IP Professional
- Industry Leading Data Capabilities
- Powerful AI technology
- Patent DNA Extraction
Browse by: Latest US Patents, China's latest patents, Technical Efficacy Thesaurus, Application Domain, Technology Topic, Popular Technical Reports.
© 2024 PatSnap. All rights reserved.Legal|Privacy policy|Modern Slavery Act Transparency Statement|Sitemap|About US| Contact US: help@patsnap.com